How to Start a Career in CyberSecurity?
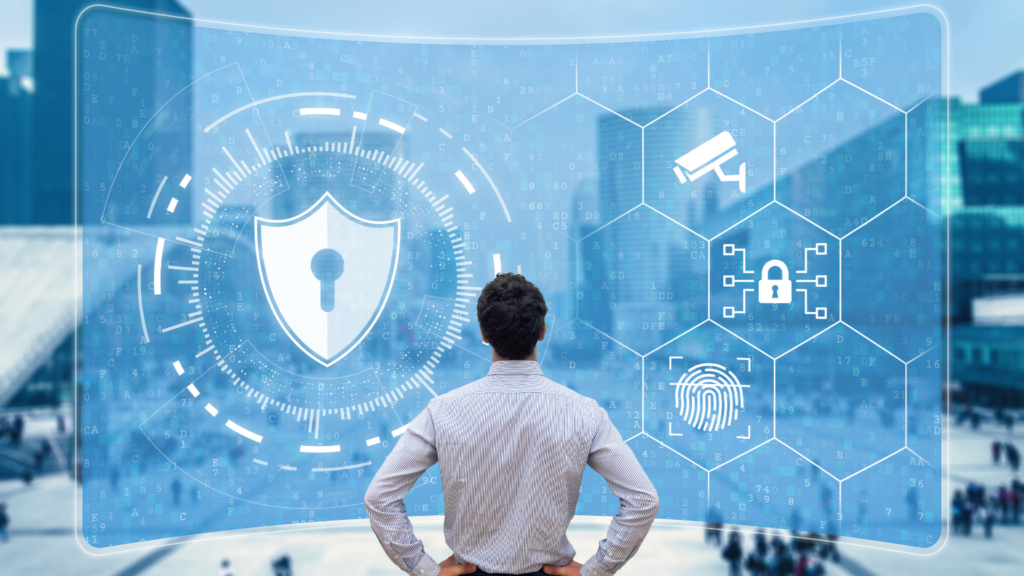
Cybersecurity is a rapidly growing field with a high demand for professionals. With the increasing number of cyber attacks, companies are looking for skilled individuals to protect their data and systems. If you are interested in starting a career in cybersecurity and wondering How to Start a Career in CyberSecurity? This guide will provide you with all the information you need to get started. Data Warehouse vs Data Mart: A Detailed Comparison CyberSecurity and it’s Importance Cybersecurity is the practice of protecting computer systems, networks, and sensitive information from unauthorized access, theft, or damage. Cybersecurity professionals use various techniques and tools to prevent cyber attacks and protect against potential threats. Cybersecurity is important because it protects sensitive information from being accessed by unauthorized individuals. Cyber attacks can result in data breaches, financial loss, and damage to a company’s reputation. Cybersecurity professionals play a critical role in preventing these attacks and ensuring the safety of sensitive information. Highest Paying Jobs in CyberSecurity According to the Cybersecurity Jobs Report, the highest paying jobs in cybersecurity include: How to Start a Career in CyberSecurity? 1. Gain Relevant Education and Certifications To start a career in cybersecurity, you need to have a strong educational background. A degree in computer science, information technology, or cybersecurity is highly valued in the industry. Additionally, certifications such as Certified Information Systems Security Professional (CISSP), Certified Ethical Hacker (CEH), and CompTIA Security+ are highly valued by employers. 2. Gain Relevant Experience Experience is crucial in the cybersecurity industry. You can gain experience through internships, entry-level positions, or volunteer work. It is important to gain experience in areas such as network security, information security, and cybersecurity operations. 3. Build a Strong Network Networking is important in any industry, and cybersecurity is no exception. Attend industry events, join cybersecurity groups, and connect with professionals on LinkedIn. Building a strong network can help you learn about job opportunities and gain valuable insights into the industry. 4. Create a Strong Resume A strong resume is essential in getting hired in cybersecurity. Your resume should highlight your education, certifications, and relevant experience. Additionally, it should be tailored to the specific job you are applying for. 5. Prepare for Interviews Preparing for interviews is crucial in getting hired in cybersecurity. Research the company and the position you are applying for, and prepare answers to common interview questions. Additionally, be prepared to discuss your experience and how it relates to the job you are applying for. How to Stand Out in CyberSecurity? 1. Stay Up-to-Date with the Latest Trends and Technologies The cybersecurity industry is constantly evolving, and it is important to stay up-to-date with the latest trends and technologies. Attend industry events, read industry publications, and participate in online forums to stay informed. 2. Develop Specialized Skills Developing specialized skills can help you stand out in the cybersecurity industry. Specialized skills such as penetration testing, threat intelligence, and incident response are highly valued by employers. 3. Join Professional Organizations Joining professional organizations such as the International Association of Computer Security Professionals (IACSP) and the Information Systems Security Association (ISSA) can help you stay informed about the latest trends and technologies in the industry. Additionally, it can provide you with networking opportunities and access to job postings. 4. Contribute to the Industry Contributing to the industry can help you stand out in the cybersecurity industry. This can include writing articles, speaking at industry events, and participating in open-source projects. Conclusion Starting a career in cybersecurity can be challenging, but it is a rewarding and exciting field. By gaining relevant education and certifications, gaining experience, building a strong network, creating a strong resume, and preparing for interviews, you can increase your chances of getting hired in the industry. Additionally, by staying up-to-date with the latest trends and technologies, developing specialized skills, joining professional organizations, and contributing to the industry, you can stand out in the cybersecurity industry and advance your career. References
Top 10 Big Tech Companies: A Comprehensive Guide
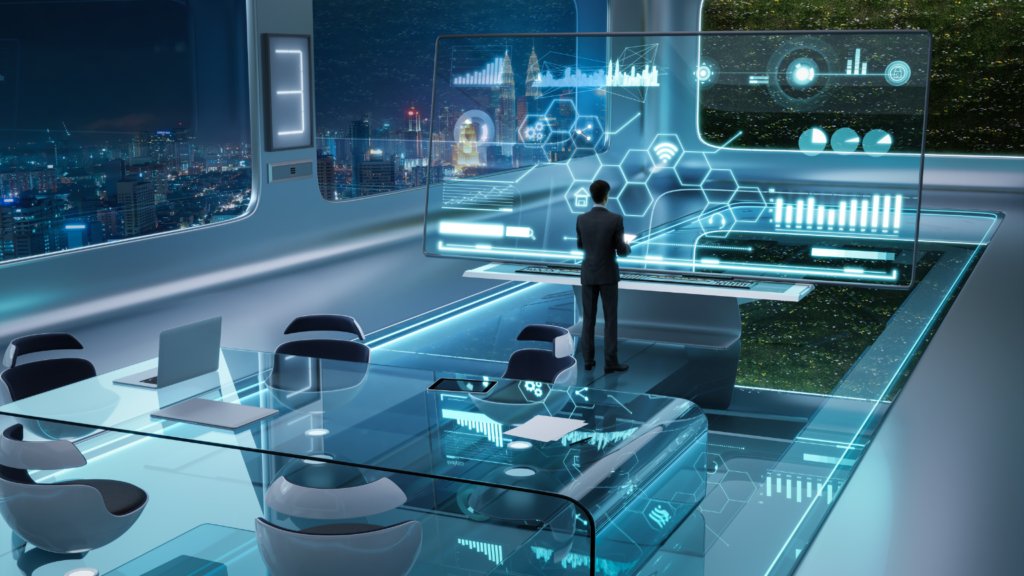
The tech industry is one of the most dynamic and innovative sectors in the world, with new companies emerging every day. However, not all tech companies are created equal. In this article, we’ll take a closer look at the top 10 big tech companies, based on data from a recent research ethics course report. Accelerate Your Career in AI with Best Machine Learning Courses Tech Industry The tech industry is a rapidly growing sector that has transformed the way we live and work. From smartphones to social media, tech companies have revolutionized the way we communicate, access information, and conduct business. In this article, we’ll explore the top 10 tech companies, based on factors such as market capitalization, revenue, and innovation. Factors to Consider When evaluating tech companies, there are several factors to consider. These include: Data Warehouse vs Data Mart: A Detailed Comparison Top 10 Big Tech Companies Based on these factors, here are the top 10 tech companies: Conclusion The tech industry is a dynamic and innovative sector that offers a range of exciting job opportunities. Based on data from a recent research ethics course we have identified the top 10 tech companies based on different factors. While these companies have made significant contributions to the tech industry, they also face challenges related to ethical AI development and data privacy. For example, many of these companies have been criticized for their handling of user data and their potential impact on democracy and social justice. Despite these challenges, the tech industry continues to grow and evolve, with new companies and technologies emerging every day. As we move forward, it’s important to prioritize ethical AI development and data privacy, while also embracing the opportunities that technology can offer. References
Exploring the Best Software Engineering Boot Camps of 2023
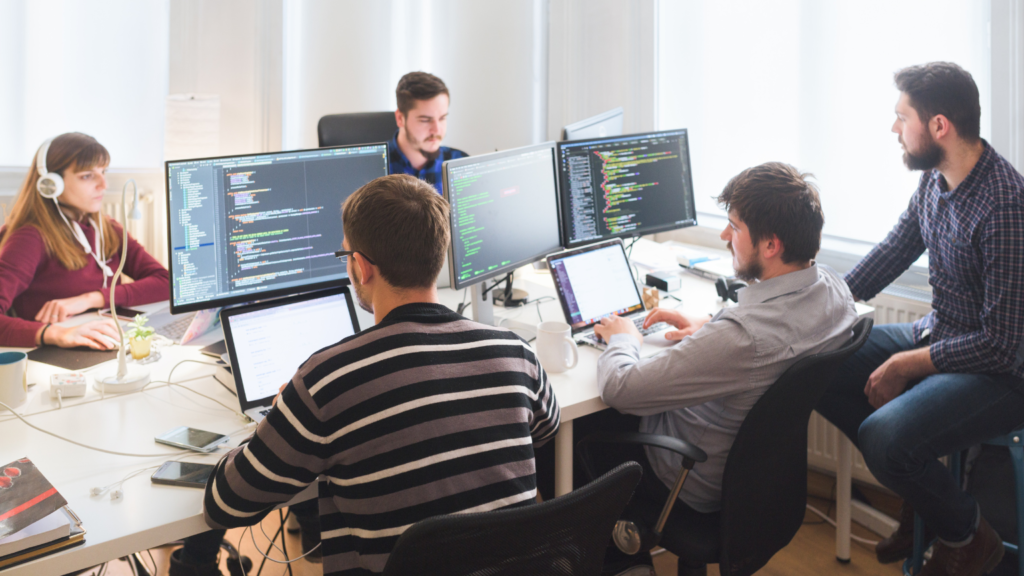
Are you looking to jumpstart your career in software engineering? A coding bootcamp may be the perfect solution for you. These intensive programs offer a fast-track to learning the skills needed to become a software engineer, with many boasting high job placement rates and partnerships with top tech companies. In this article, we’ll take a closer look at the top 10 online software engineering bootcamps of 2023. Tpu vs Gpu: The Giants of Computational Power Top 10 Software Engineering Boot Camps of 2023 1. General Assembly Immersive Courses are available both online and in-person, and offer a comprehensive curriculum covering everything from web development to data structures and algorithms. With a tuition cost of $15,950, this bootcamp is on the pricier side, but it boasts an impressive list of hiring partners including Chase, Adobe, Google, Facebook, IBM, DirectTV, Microsoft, CocaCola, Samsung, and PlayStation. Pros: Comprehensive curriculum, impressive list of hiring partners Cons: High tuition cost 2. Flatiron School This Bootcamp is available both online and in-person, with a tuition cost of $15,000. The curriculum covers full-stack web development, computer science fundamentals, and more. Flatiron School’s hiring partners include Google, Microsoft, and more. Pros: Comprehensive curriculum, flexible format options Cons: High tuition cost, may not have as many hiring partners as some other bootcamps. 3. App Academy It is a full-time, online-only program with a tuition cost of $17,000. The curriculum covers full-stack web development, computer science fundamentals, and more. App Academy’s hiring partners include Google, Microsoft, and more. Pros: Comprehensive curriculum, high job placement rate Cons: High tuition cost, full-time commitment may not be suitable for all learners 4. Hack Reactor It is a full-time, online-only program with a tuition cost of $17,980. The curriculum covers full-stack web development, computer science fundamentals, and more. Hack Reactor’s hiring partners include Amazon, Google, Microsoft, and more. Pros: Comprehensive curriculum, high job placement rate Cons: High tuition cost, full-time commitment may not be suitable for all learners 5. Thinkful It is an online-only program with a tuition cost of $16,000. The curriculum covers full-stack web development, data structures and algorithms, and more. Thinkful’s hiring partners include Google, IBM, and more. Pros: Comprehensive curriculum, high job placement rate Cons: High tuition cost, online-only format may not be suitable for all learners 6. Springboard It is an online-only program with a tuition cost ranging from $9,900 to $16,500. The curriculum covers full-stack web development, data structures and algorithms, and more. Springboard’s hiring partners include Ford, Facebook, CapitalOne, Verizon, Microsoft, Amazon, Oracle, J.P Morgan, Boeing, State Farm, American Express, Dell, Bank of America, Lockheed Martin, and Apple. Pros: Flexible tuition options, impressive list of hiring partners Cons: Online-only format may not be suitable for all learners 7. Fullstack Academy It is available both online and in-person, with a tuition cost of $17,910. The curriculum covers full-stack web development, computer science fundamentals, and more. Fullstack Academy’s hiring partners include Google, Microsoft, and more. Pros: Comprehensive curriculum, flexible format options Cons: High tuition cost, may not have as many hiring partners as some other bootcamps 8. Coding Dojo Coding Dojo’s Software Engineering Bootcamp is available both online and in-person, with a tuition cost of $15,995. The curriculum covers full-stack web development, computer science fundamentals, and more. Coding Dojo’s hiring partners include Amazon, Microsoft, and more. Pros: Comprehensive curriculum, flexible format options Cons: High tuition cost, may not have as many hiring partners as some other bootcamps 9. CareerFoundry CareerFoundry’s Software Engineering Bootcamp is an online-only program with a tuition cost of $6,900. The curriculum covers full-stack web development, computer science fundamentals, and more. CareerFoundry’s hiring partners include Google, Microsoft, and more. Pros: Affordable tuition, comprehensive curriculum Cons: May not have as many hiring partners as some other bootcamps 10. BrainStation It can be completed through live online classes or in person. The program covers full-stack development, including front-end and back-end technologies, and includes a final project that allows students to showcase their skills to potential employers. BrainStation also offers career services to help graduates find job opportunities after completing the program. Pros: BrainStation provides hands-on, industry-relevant coding training. Cons: It’s costly and less comprehensive than traditional degrees. Conclusion Choosing the right software engineering bootcamp can be a daunting task, but with the information provided in this article, you can make an informed decision. Whether you’re looking for a comprehensive curriculum, flexible format options, or impressive hiring partners, there is a bootcamp on this list that will meet your needs. Keep in mind that while tuition costs may be high, the potential return on investment in terms of job placement and earning potential can make it well worth it. Be sure to carefully consider your future goals and the specific offerings of each bootcamp before making your final decision. With the right bootcamp, you can take the first step towards a successful career in software engineering.
Git Rebase Vs Merge: A Detailed Insight
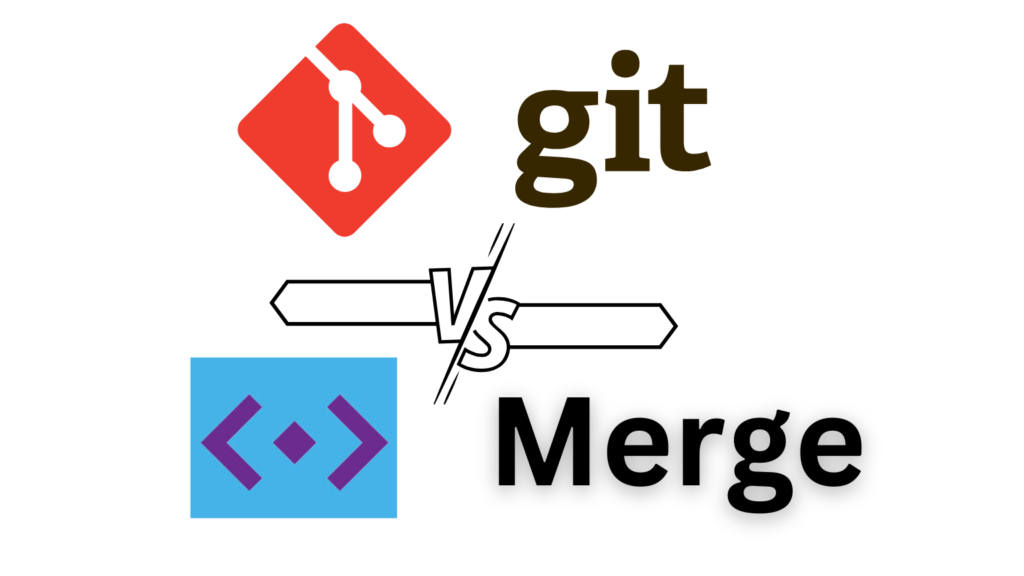
Git is a powerful tool for version control that allows developers to manage their code changes and collaborate with others. One of the most important features of Git is the ability to merge changes from one branch into another. However, there is another option called rebase that can also be used to integrate changes. In this article, we will explore the differences between Git rebase vs merge and when to use each one. What is Git Merge? Git merge is a command that allows you to combine changes from one branch into another. When you merge a branch, Git creates a new commit that incorporates the changes from both branches. This creates a new point in the Git history where the two branches have been merged. Merging is a simple and straightforward way to integrate changes into your codebase. It is especially useful when you are working on a team and need to combine changes from multiple developers. However, merging can also create a messy Git history, especially if there are conflicts that need to be resolved. What is Git Rebase? Git rebase is another way to integrate changes from one branch into another. However, instead of creating a new commit that merges the changes, rebase rewrites the Git history to make it look like the changes were made on the same branch. When you rebase a branch, Git takes the changes from the branch you are rebasing onto and applies them to your branch. This creates a linear Git history where all the changes appear to have been made on the same branch. Rebasing can be a powerful tool for keeping your Git history clean and easy to understand. It can also be useful when you are working on a feature branch and want to incorporate changes from the main branch without creating a merge commit. JSON vs CSV: A Comparative Analysis of Data Formats Git Rebase Vs Merge: Key Differences Now that we understand what Git merge and rebase are, let’s take a closer look at the differences between them. 1. Git Merge Creates a Merge Commit, Git Rebase Does Not When you merge a branch in Git, Git creates a new commit that combines the changes from both branches. This is called a merge commit. Merge commits can be useful for keeping track of when changes were merged and for resolving conflicts. When you rebase a branch in Git, Git does not create a merge commit. Instead, it applies the changes from the other branch directly to your branch. This creates a linear Git history where all the changes appear to have been made on the same branch. Git Merge Preserves Branching History, Git Rebase Does Not When you merge a branch in Git, Git preserves the branching history of the project. This means that you can see when a branch was created, when it was merged, and where it was merged into. This can be useful for understanding the development history of a project. When you rebase a branch in Git, Git does not preserve the branching history of the project. Instead, it makes it look like all the changes were made on the same branch. This can make it more difficult to understand the development history of a project. Git Rebase Can Create a Cleaner Git History Because Git rebase does not create merge commits, it can create a cleaner Git history. This can make it easier to understand the development history of a project and can make it easier to find specific changes. However, it is important to note that rebasing can also create problems if not done correctly. If you rebase a branch that has already been pushed to a remote repository, it can cause problems for other developers who are working on the same branch. Keras vs PyTorch: An In-depth Comparison and Understanding When to Use Git Merge Git merge is a good option when you want to preserve the branching history of a project. It is also a good option when you are working on a team and need to combine changes from multiple developers. Merge can also be useful when you are working on a branch that has already been pushed to a remote repository. Because merge creates a new commit, it does not change the Git history of the project and does not cause problems for other developers. When to Use Git Rebase Git rebase is a good option when you want to create a cleaner Git history. It can also be useful when you are working on a feature branch and want to incorporate changes from the main branch without creating a merge commit. Rebasing can also be useful when you are working on a branch that has not yet been pushed to a remote repository. Because rebasing changes the Git history of the project, it is important to avoid rebasing branches that have already been pushed to a remote repository. Conclusion Git merge and rebase are both powerful tools for integrating changes in a Git repository. While merge preserves the branching history of a project, rebase can create a cleaner Git history. The choice between merge and rebase depends on the specific needs of your project. When working on a team, it is important to establish guidelines for when to use merge and when to use rebase. This can help ensure that the Git history of the project remains clean and easy to understand for all developers. In general, it is a good idea to use merge for branches that have already been pushed to a remote repository and for branches that have a complex branching history. Use rebase for feature branches and for branches that have not yet been pushed to a remote repository. By understanding the differences between Git merge and rebase, you can make informed decisions about how to integrate changes in your Git repository. Whether you choose merge or rebase, the most important thing is to maintain a clean and understandable Git
OpenShift Vs Kubernetes: A Comprehensive Comparison
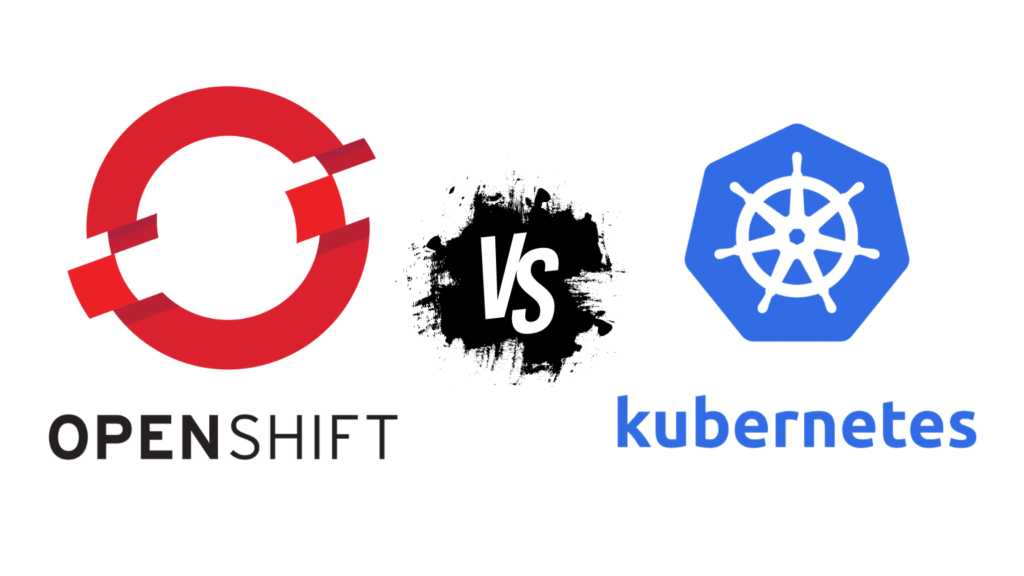
Containerization has become a popular way to deploy and manage applications in the cloud. With containerization, developers can package their applications and dependencies into a single unit that can run consistently across different environments. However, managing containers at scale can be challenging, which is where container orchestration tools like OpenShift and Kubernetes come in. In this article, we will compare OpenShift vs Kubernetes to help you decide which one is better for your needs. Keras vs PyTorch: An In-depth Comparison and Understanding Understanding Kubernetes Kubernetes is an open-source container orchestration tool that was originally developed by Google. It provides a platform for automating the deployment, scaling, and management of containerized applications. Kubernetes has a large and active community of contributors, which has helped it become the de facto standard for container orchestration. Understanding OpenShift OpenShift is a container application platform that is built on top of Kubernetes. It provides additional features and tools to make it easier to deploy, manage, and scale containerized applications. OpenShift is developed and maintained by Red Hat, a leading provider of open-source software solutions. OpenShift Vs Kubernetes Architecture Both OpenShift and Kubernetes are based on a similar architecture. They use a master-slave architecture, where the master node is responsible for managing the cluster and the worker nodes are responsible for running the containers. However, OpenShift provides additional features on top of Kubernetes, such as integrated CI/CD pipelines, built-in monitoring, and logging. Ease of Use OpenShift is designed to be easy to use, even for developers who are new to containerization. It provides a web-based console that allows you to manage your applications and infrastructure from a single interface. OpenShift also provides a number of pre-built templates and workflows that make it easy to get started with containerization. Kubernetes, on the other hand, has a steeper learning curve. It requires a deeper understanding of containerization and networking concepts. However, Kubernetes provides more flexibility and control over your infrastructure, which can be beneficial for more complex applications. Scalability Both OpenShift and Kubernetes are designed to be highly scalable. They can scale up or down based on the demand for your applications. However, OpenShift provides additional features to make it easier to scale your applications. For example, it provides automatic load balancing and horizontal scaling, which can help you handle sudden spikes in traffic. Security Security is a critical concern when it comes to containerization. Both OpenShift and Kubernetes provide a number of security features to help protect your applications. For example, they both provide role-based access control, network policies, and container isolation. However, OpenShift provides additional security features, such as integrated image scanning and compliance reporting, which can help you identify and address security vulnerabilities in your applications. JSON vs CSV: A Comparative Analysis of Data Formats Community Support Kubernetes has a large and active community of contributors, which has helped it become the de facto standard for container orchestration. This means that there are many resources available for learning and troubleshooting Kubernetes. OpenShift also has a community of contributors, but it is smaller than Kubernetes. However, OpenShift provides premium support options, which can be beneficial for organizations that require additional support. Infrastructure Support Both OpenShift and Kubernetes can be hosted on bare metal servers or in the cloud. They both support all major cloud providers, such as AWS, GCP, and Azure. However, Kubernetes requires some custom configuration, such as ingresses, that needs to be set up even when using managed services. OpenShift has its own pre-configured routes for routing public traffic to inside the containers. Cost Both OpenShift and Kubernetes are open-source tools, which means that they are free to use. However, there may be additional costs associated with using these tools, such as hosting fees, support costs, and licensing fees for third-party tools. OpenShift provides a subscription model, where the cost increases with the complexity of your infrastructure. Kubernetes can be used with managed services from cloud providers, which can help reduce costs. Conclusion oth OpenShift and Kubernetes are powerful container orchestration tools that can help you deploy, manage, and scale containerized applications. However, they have different strengths and weaknesses, which make them better suited for different use cases. OpenShift is designed to be easy to use and provides additional features for security and scalability. Kubernetes provides more flexibility and control over your infrastructure, but has a steeper learning curve. Ultimately, the choice between OpenShift and Kubernetes depends on your specific needs and requirements.
AWS vs Azure: A Comprehensive Comparison of Cloud Services
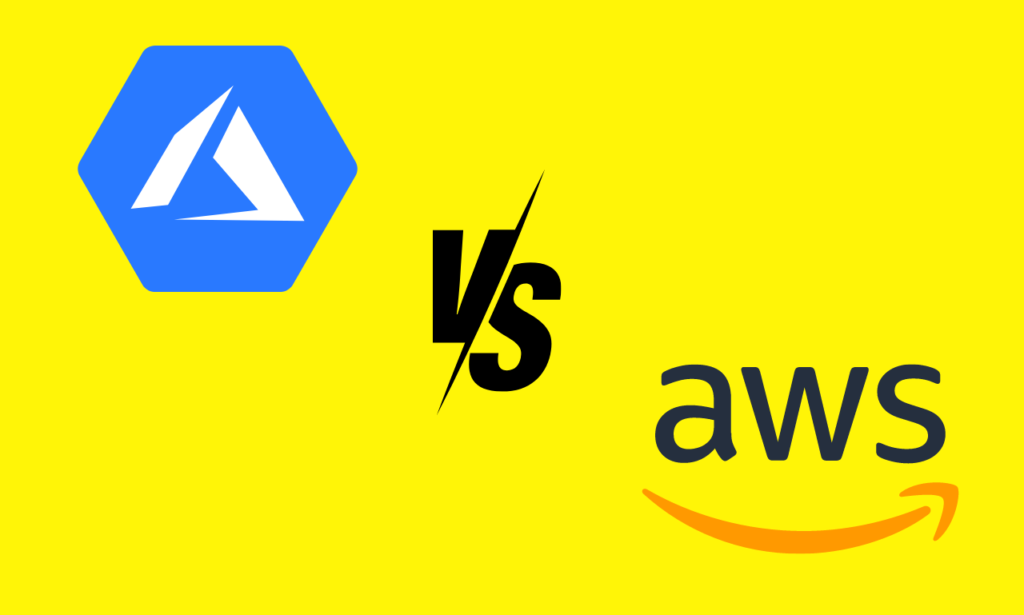
Cloud computing has revolutionized the way businesses operate, providing a flexible and scalable infrastructure that can be accessed from anywhere in the world. Two of the biggest players in the cloud market are Amazon Web Services (AWS) and Microsoft Azure. In this article, we’ll take a deep dive into the features and capabilities of these two cloud services, and compare them to help you determine which one is best suited for your organization’s needs. AWS vs Azure AWS is a cloud computing platform offered by Amazon, providing a wide range of services including computing, storage, and databases. It was launched in 2006 and has since become the market leader in cloud computing, with a 32% share of the market as of 2022. Azure, on the other hand, is a cloud computing platform offered by Microsoft, providing similar services to AWS. It was launched in 2010 and has since grown to become the second-largest cloud provider, with a 23% share of the market as of 2022. Keras vs PyTorch: An In-depth Comparison and Understanding Pricing One of the most important factors to consider when choosing a cloud provider is pricing. Both AWS and Azure offer a pay-as-you-go pricing model, which means you only pay for the resources you use. However, the pricing structures of the two providers differ slightly. AWS offers a wide range of pricing options, including on-demand, reserved instances, and spot instances. Azure also offers a range of pricing options, including pay-as-you-go, reserved instances, and spot instances. However, Azure’s reserved instances are more flexible than AWS’s, allowing you to change the instance type or region at any time without penalty. Azure also offers a hybrid benefit program, which allows you to use your existing licenses to save more. Compute Services Both AWS and Azure offer a wide range of compute services, including virtual machines, containers, and serverless computing. Virtual Machines: AWS offers a wide range of virtual machine options, including general-purpose, memory-optimized, and compute-optimized instances. Azure offers similar options, including general-purpose, memory-optimized, and compute-optimized instances. Both providers offer a variety of operating systems and support for popular programming languages. Containers: AWS offers Amazon Elastic Container Service (ECS) and Amazon Elastic Kubernetes Service (EKS) for container management. Azure offers Azure Container Instances and Azure Kubernetes Service (AKS). Both providers offer support for popular container orchestration tools like Docker and Kubernetes. Serverless Computing: AWS offers AWS Lambda for serverless computing, which allows you to run code without provisioning or managing servers. Azure offers Azure Functions, which provides similar functionality. Both providers offer support for a wide range of programming languages and integrations with other services. Both AWS and Azure offer a wide range of storage services, including object storage, block storage, and file storage. Object Storage: AWS offers Amazon S3 for object storage, which is highly scalable and durable. Azure offers Azure Blob Storage, which provides similar functionality. Both providers offer support for lifecycle policies, versioning, and encryption. Block Storage: AWS offers Elastic Block Store (EBS) for block storage, which provides high-performance storage for virtual machines. Azure offers Azure Disk Storage, which provides similar functionality. Both providers offer support for different disk types and encryption. File Storage: AWS offers Amazon Elastic File System (EFS) for file storage, which provides scalable and highly available file storage. Azure offers Azure Files, which provides similar functionality. Both providers offer support for different file systems and encryption. JSON vs CSV: A Comparative Analysis of Data Formats Big Data Services Both AWS and Azure offer a wide range of big data services, including data warehousing, analytics, and machine learning. Data Warehousing: AWS offers Amazon Redshift for data warehousing, which provides petabyte-scale data warehousing. Azure offers Azure SQL Data Warehouse, which provides similar functionality. Both providers offer support for different data sources and integrations with other services. Analytics: AWS offers Amazon EMR for big data analytics, which provides a managed Hadoop framework. Azure offers Azure HDInsight, which provides similar functionality. Both providers offer support for different analytics tools and integrations with other services. Machine Learning: AWS offers Amazon SageMaker for machine learning, which provides a fully managed platform for building, training, and deploying machine learning models. Azure offers Azure Machine Learning, which provides similar functionality. Both providers offer support for different machine learning frameworks and integrations with other services. Conclusion Both AWS and Azure offer a wide range of cloud services with similar pricing models and features. AWS is the market leader in cloud computing, with a wider range of services and a more mature platform. Azure, on the other hand, offers better integration with Microsoft’s on-premises technologies and a more consistent user interface. When choosing between AWS and Azure, it’s important to consider your organization’s specific needs and requirements. If you’re already using Microsoft technologies, Azure may be the better choice for you. If you need a wider range of services and a more mature platform, AWS may be the better choice. Ultimately, both AWS and Azure are excellent choices for cloud computing, and the decision between the two will depend on your organization’s specific needs and priorities. By considering the factors we’ve discussed in this article, you can make an informed decision and choose the cloud provider that’s right for you.
Inferential Statistics vs Descriptive Statistics: A Comparative Study
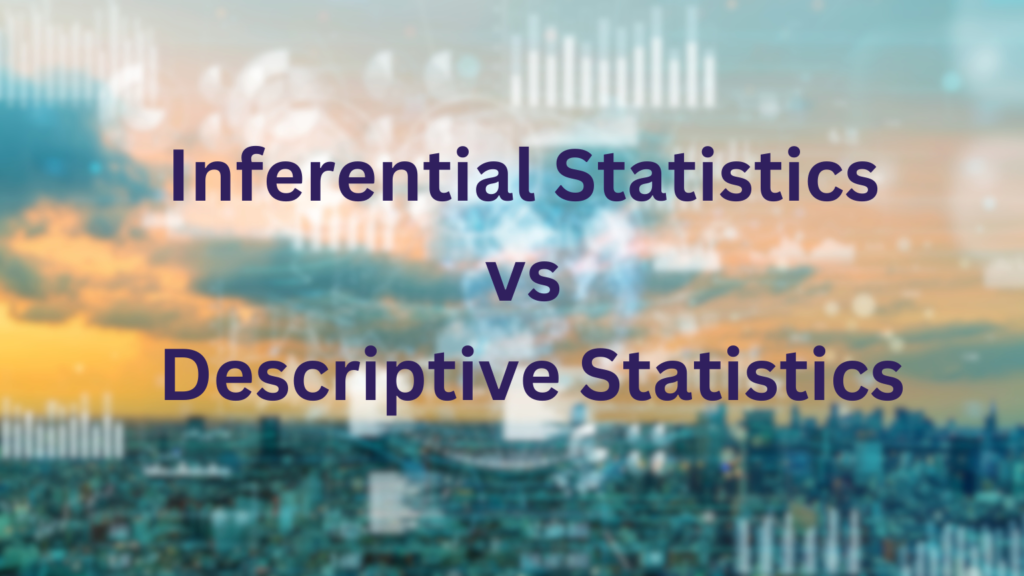
Statistics is a crucial aspect of data analysis, and it is used to make sense of large amounts of data. There are two main types of statistics: Inferential Statistics vs Descriptive Statistics. In this article, we will explore the differences between these two types of statistics and their measurement techniques. What is Descriptive Statistics? Descriptive statistics is a technique used to describe and summarize data in a meaningful way. It is a simple technique that involves choosing a group of interest, recording data about the group, and then using summary statistics and graphs to describe the group properties. Descriptive statistics is used to describe the characteristics of a data set, and there is no uncertainty involved because you are just describing the people or items that you actually measure. Measurement Techniques Used in Descriptive Statistics There are several measurement techniques used in descriptive statistics, including: What is Inferential Statistics? Inferential statistics is a technique used to draw conclusions or generalizations about a broader population using a sample of data. It involves using probability theory to make inferences about a population based on a sample. Inferential statistics is used when you want to know if there is a significant difference in the outcomes of patients who receive a drug compared to those who receive a placebo if you want to know if a new drug is effective. Visual Studio Code vs Visual Studio What is an example of an Inferential Statistic? Let’s revisit the school scenario. Suppose the school wants to understand if a new teaching method has improved students’ performance. They could implement the method with a small group, then use inferential statistics to predict if the method would have a similar impact on the larger student body. This predictive capacity is a key aspect of inferential statistics. What is Meant by Inferential Statistics? Inferential statistics involves making predictions or drawing conclusions about a population based on observations and analyses of a sample. It uses methods such as hypothesis testing, regression analysis, and variance analysis to make predictions about unknown population parameters. Measurement Techniques Used in Inferential Statistics There are several measurement techniques used in inferential statistics, including: Keras vs PyTorch: An In-depth Comparison and Understanding The Impact of Inferential Statistics vs Descriptive Statistics on Data Analytics Descriptive and inferential statistics are both important in data analytics. Descriptive statistics is used to describe the characteristics of a data set, and it is useful in identifying patterns and trends in data. It is often used in exploratory data analysis to gain insights into the data before moving on to more complex analyses. Descriptive statistics is also useful in summarizing data in a way that is easy to understand and communicate to others. Inferential statistics, on the other hand, is used to make predictions or generalizations about a population based on a sample of data. It is useful in hypothesis testing and in making decisions based on data. Inferential statistics is also used in predictive modeling, where the goal is to predict future outcomes based on past data. Both descriptive and inferential statistics are important in data analytics because they help us make sense of large amounts of data. They are used to identify patterns and trends, make predictions, and make decisions based on data. Without these statistical techniques, it would be difficult to draw meaningful conclusions from data. Conclusion Descriptive and inferential statistics are two important statistical techniques used in data analytics. Descriptive statistics is used to describe and summarize data, while inferential statistics is used to make predictions or generalizations about a population based on a sample of data. Both techniques are important in data analytics because they help us make sense of large amounts of data and draw meaningful conclusions. By understanding the differences between these two techniques and their measurement techniques, we can use them effectively in our data analysis. References
Snowflake vs Star Schema: A Detailed Comparison
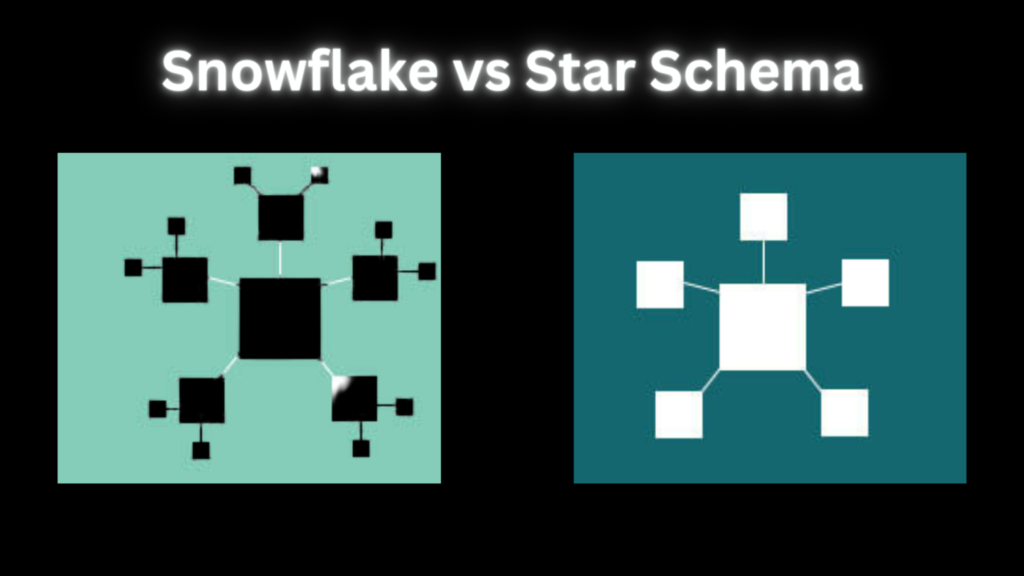
Data warehousing is an essential aspect of modern businesses, and it involves the collection, storage, and analysis of large amounts of data. To achieve this, data modeling techniques such as Snowflake vs Star Schema are commonly used. In this article, we will provide a comprehensive comparison of these two data modeling techniques, highlighting their advantages, disadvantages, and practical applications. Visual Studio Code vs Visual Studio Introduction to Star Schema and Snowflakes Schema Star Schema and Snowflakes Schema are two commonly used data modeling techniques in data warehousing. Star Schema is a simple and intuitive approach that involves a central fact table surrounded by dimension tables. The fact table contains the measures or metrics of interest, while the dimension tables provide context for the measures. Snowflakes Schema, on the other hand, is a more complex approach that involves a central fact table surrounded by dimension tables that are further normalized into sub-dimension tables. Advantages of Star Schema Star Schema has several advantages that make it a popular choice for data warehousing. Disadvantages of Star Schema Despite its advantages, Star Schema has some limitations that businesses should be aware of. Practical Applications of Star Schema Star Schema is commonly used in business intelligence applications such as sales analysis, financial reporting, and customer relationship management. For example, a sales analysis application may use a fact table containing sales data and dimension tables containing information about products, customers, and time periods. This allows businesses to analyze sales data by product, customer, and time period, providing valuable insights into sales trends and customer behavior. Data Warehouse vs Data Mart: A Detailed Comparison Advantages of Snowflakes Schema Snowflakes Schema has several advantages that make it a popular choice for complex data models. Disadvantages of Snowflakes Schema Despite its advantages, Snowflakes Schema has some limitations that businesses should be aware of. Firstly, it can result in slower query performance since it involves more joins to retrieve data. Secondly, it can be more complex to understand and implement, making it less suitable for small to medium-sized businesses. Finally, it can result in more complex maintenance since any changes to the schema require updating the sub-dimension tables as well as the dimension tables. Practical Applications of Snowflakes Schema Snowflakes Schema is commonly used in data models that require multiple levels of hierarchy, such as product catalogs, organizational charts, and geographical data. For example, a product catalog may use a fact table containing sales data and dimension tables containing information about products, categories, and suppliers. The category dimension table may be further normalized into sub-dimension tables containing information about subcategories and sub-subcategories, allowing businesses to analyze sales data at multiple levels of granularity. Comparison of Snowflake vs Star Schema When deciding between Star Schema and Snowflakes Schema, businesses should consider several factors, including the nature of their data, the complexity of their data model, and their query performance requirements. Star Schema is ideal for simple data models that require fast query performance, while Snowflakes Schema is more suitable for complex data models that require multiple levels of hierarchy. In terms of query performance, Star Schema is generally faster than Snowflakes Schema since it involves fewer joins to retrieve data. However, Snowflakes Schema can provide more flexibility in data modeling and reduce data redundancy, making it a better choice for complex data models. Maintenance is another factor to consider when choosing between Star Schema and Snowflakes Schema. Star Schema is easier to maintain since any changes to the schema only require updating the dimension tables. Snowflakes Schema, on the other hand, can be more complex to maintain since any changes to the schema require updating the sub-dimension tables as well as the dimension tables. Conclusion Star Schema and Snowflakes Schema are two commonly used data modeling techniques in data warehousing. While Star Schema is ideal for simple data models that require fast query performance, Snowflakes Schema is more suitable for complex data models that require multiple levels of hierarchy. Businesses should consider several factors, including the nature of their data, the complexity of their data model, and their query performance requirements when deciding between these two data modeling techniques. References
Best Programming Language for Data Science
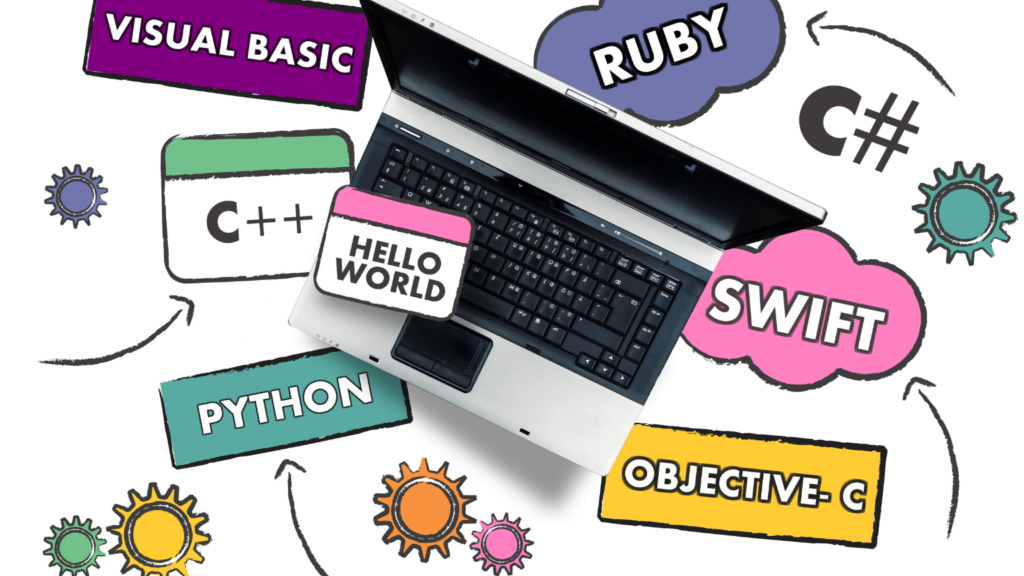
This article provides a list of the top programming languages for data science. The five best programming language for data science, are: The R Programming Language, Python Programming Language, MATLAB Programming Language, Hadoop Programming Language, and The SQL Programming Language. These programming languages are popular among data scientists for their ability to handle large datasets, their powerful data analysis capabilities, and their ease of use. Each language has its own strengths and weaknesses, and the choice of language will depend on the specific needs of the project or organization. 1. The R Programming Language: R is a free, open-source programming language that is widely used for statistical computing and graphics. It has a large and active community of users and developers, and offers a wide range of statistical and graphical techniques. R is particularly popular among data scientists for its ability to handle large datasets and its powerful data visualization capabilities. 2. Python Programming Language: Python is a general-purpose programming language that is widely used in data science, machine learning, and artificial intelligence. It is known for its simplicity, readability, and ease of use, and has a large and active community of users and developers. Python offers a wide range of libraries and frameworks for data analysis, including NumPy, Pandas, and Scikit-learn. 3. MATLAB Programming Language: MATLAB is a proprietary programming language that is widely used in scientific computing and engineering. It offers a wide range of tools and functions for data analysis, including statistical analysis, signal processing, and image processing. MATLAB is particularly popular among data scientists for its ability to handle large datasets and its powerful visualization capabilities. 4. Hadoop Programming Language: Hadoop is a distributed computing framework that is widely used for processing large datasets. It is based on the MapReduce programming model, which allows users to write programs that can process large amounts of data in parallel across a cluster of computers. Hadoop is particularly popular among data scientists for its ability to handle large datasets and its scalability. 5. The SQL Programming Language: SQL (Structured Query Language) is a programming language that is widely used for managing and querying relational databases. It offers a wide range of tools and functions for data analysis, including data aggregation, filtering, and sorting. SQL is particularly popular among data scientists for its ability to handle large datasets and its ease of use. Data Warehouse vs Data Mart: A Detailed Comparison Conclusion The choice of language will depend on the specific needs of the project or organization. It is recommended to evaluate the requirements of the project and the strengths of each language before making a decision on which language to use. Visual Studio Code vs Visual Studio References
GPT 4 vs GPT 3: The Evolution of Language Models
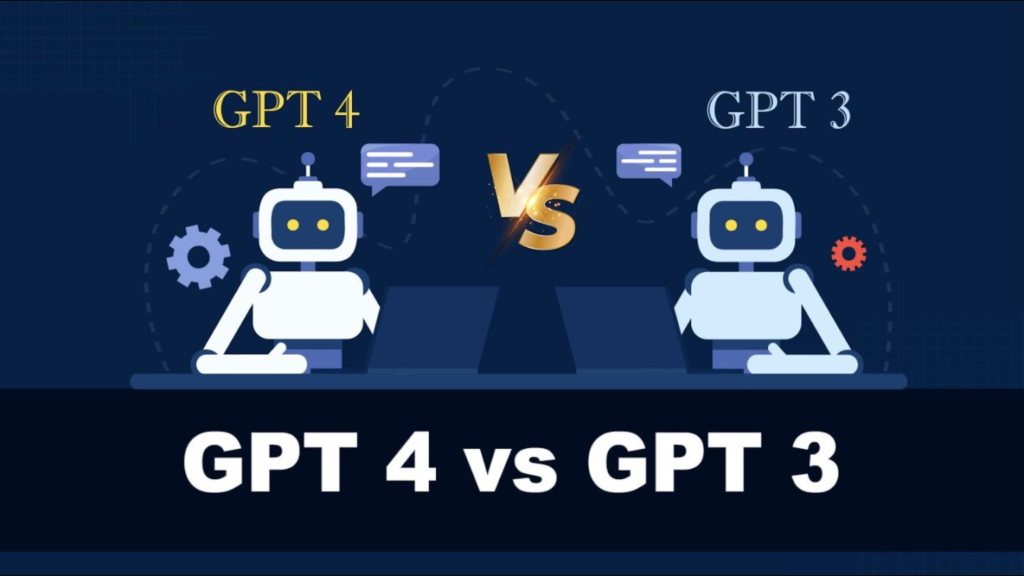
In recent years, the field of Natural Language Processing (NLP) has seen significant advancements, with the development of Generative Pre-trained Transformer (GPT) models. These models have revolutionized the way we interact with machines, enabling them to understand and generate human-like language. In this article, we will explore the differences between GPT-4 and GPT-3, two of the most advanced NLP models to date. Introduction to GPT-3 and GPT-4 GPT-3, released in 2020, is the third iteration of the GPT series, developed by OpenAI. It is a language model that uses deep learning techniques to generate human-like text. GPT-3 has a context window of 2048 and can generate up to 3000 words of text. It has been widely used in various NLP tasks, including language translation, question-answering, and text completion. GPT-4, on the other hand, is the latest addition to the GPT series, and it promises to be even more advanced than its predecessor. While the technical details of GPT-4 are not yet available, it is expected to have a larger context window and generate longer text than GPT-3. GPT 4 vs GPT 3: A Comprehensive Comparison Context Window One of the significant differences between GPT-3 and GPT-4 is the context window size. The context window refers to the number of words or tokens that the model can take as input. GPT-3 has a context window of 2048, while GPT-4 has a context window of up to 32768, depending on the version. This means that GPT-4 can take in 4 to 16 times more input than GPT-3, allowing it to process more information and generate more accurate and relevant responses. Visual Studio Code vs Visual Studio Output Length Another significant difference between GPT-3 and GPT-4 is the length of the generated text. GPT-3 can generate up to 3000 words of text, while GPT-4 can generate up to 24000 words, which is equivalent to 48 pages. This is eight times more than what GPT-3 can generate, constrained by 3000 words, which is equivalent to 6 pages. This means that GPT-4 can generate longer and more detailed responses, making it more suitable for tasks that require generating lengthy text. Performance GPT-4 is expected to outperform GPT-3 in various NLP tasks. GPT-3 has already demonstrated impressive performance in tasks such as language translation, question-answering, and text completion. However, GPT-4 is expected to perform even better due to its larger context window and increased model size. One of the areas where GPT-4 is expected to excel is in language translation. GPT-3 has already shown impressive performance in this area, but GPT-4 is expected to be even better due to its larger context window and improved accuracy. Another area where GPT-4 is expected to perform well is in text completion. GPT-3 has already shown impressive performance in this area, but GPT-4 is expected to generate more accurate and relevant responses due to its larger context window and increased model size. Limitations and Challenges While GPT-4 promises to be a significant advancement in the field of NLP, it also comes with its limitations and challenges. One of the significant challenges is the increased processing and computing resource requirements. GPT-4’s larger model size and context window require more significant processing power and computing resources, which can be a challenge for some organizations. Another challenge is the potential for malicious usage of the technology. GPT-4’s ability to generate human-like text can be used for nefarious purposes, such as creating fake news or impersonating individuals online. It is essential to work towards improving the safety and reliability of the technology to limit its malicious usage. Data Warehouse vs Data Mart: A Detailed Comparison Conclusion GPT-4 promises to be a significant advancement in the field of NLP, with its larger context window and increased model size. It is expected to outperform GPT-3 in various NLP tasks, including language translation and text completion. However, it also comes with its limitations and challenges, such as increased processing and computing resource requirements and the potential for malicious usage. It is essential to work towards improving the safety and reliability of the technology while also exploring ways to make it more accessible and open-source. References