Best Data Labeling Company in 2023
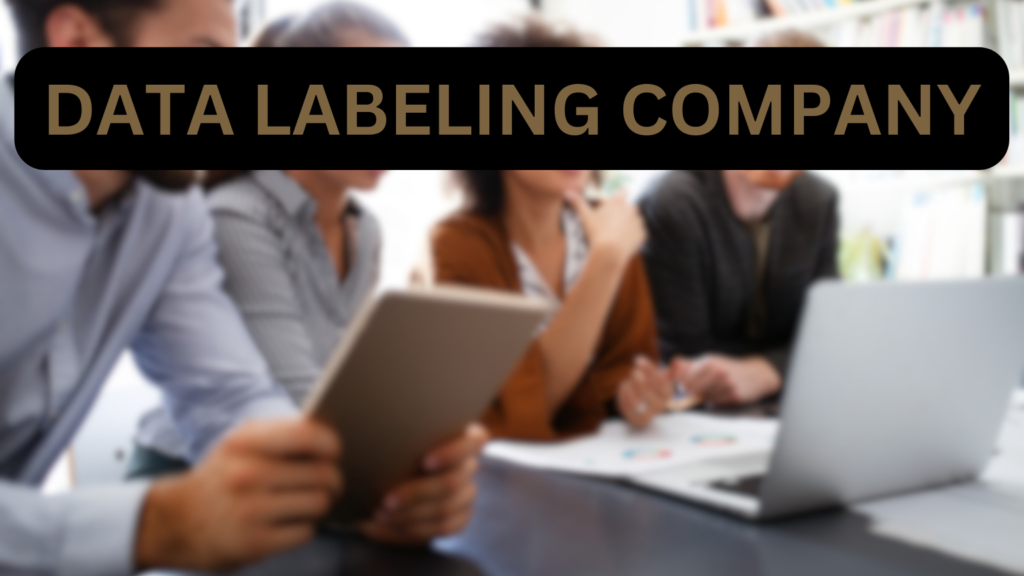
A data labeling company provides services centred on the annotation, tagging, or labeling of data. This data can come in many forms: text, images, videos, and more. These labels, often added manually by human annotators, provide context and meaning to the data. This makes it usable for machine learning algorithms and AI systems to use data. Data labeling companies primarily help to transform raw data into something more meaningful by adding relevant labels. For example, in the realm of image recognition, data labelers may identify and label objects within an image. This can enable an AI system to understand the image’s content and use it accordingly. The data thus labeled becomes training data. This is then used to teach machine learning models to predict or interpret new, unlabeled data. Top 10 Data Labeling Company 1. People for AI: Overview: Data labeling and validation platform. Features: Combines human expertise and technology, caters to various industries, and ensures high-quality labeled data. 2. SunTec AI: Overview: Provides AI data services, including collection, annotation, and validation. Features: Customizable solutions, supports multiple data types, experienced annotators. 3. CloudFactory: Overview: Combines human workforce with technology for scalable data work. Features: Managed teams, tech-forward approach, quality assurance. 4. ByteBridge: Overview: Real-time data labeling platform with automated tools. Features: ML-assisted labeling, flexibility in output formats, supports computer vision projects. 5. Label Your Data: Overview: Data annotation services for various industries. Features: Image annotation, text categorization, audio transcription. 6. Zuru Annotation Platform: Overview: Provides tools and services for quality data labeling. Features: Multiple annotation types, QA processes, supports large-scale projects. 7. Edgecase: Overview: Specializes in high-quality data enrichment. Features: Data curation tools, quality assurance, custom workflows. 8. Supahands: Overview: Outsourcing platform for businesses. Features: Task management tools, quality assurance, scalability. 9. Triyock BPO Services: Overview: Business Process Outsourcing company with various services. Features: Data entry, data labeling, quality check. 10. Clickworker: Overview: A micro-task online platform similar to Mechanical Turk. Features: Global workforce, task flexibility, diverse data tasks. Each company offers unique strengths, so the best choice will ultimately depend on your project’s specific requirements, scale, and domain. Embarking on a Career as a Data Labeler 1. Understand the role of a data labeler: A data labeler is responsible for labeling data sets to help train machine learning models. This involves identifying and categorizing data, such as images, text, or audio, and labeling them with relevant tags or annotations. 2. Develop the necessary skills: To become a data labeler, you need to have strong attention to detail, good communication skills, and the ability to work with large amounts of data. You should also have some knowledge of machine learning concepts and tools, such as Python, TensorFlow, or PyTorch. 3. Gain experience: You can gain experience by working on small projects or volunteering to label data sets for open-source projects. This will help you build your skills and develop a portfolio of work that you can showcase to potential employers. 4. Look for job opportunities: There are many job opportunities for data labelers in industries such as healthcare, finance, and technology. You can search for job openings on job boards, company websites, or through staffing agencies. 5. Prepare for interviews: When preparing for interviews, be sure to highlight your attention to detail, communication skills, and experience working with data. You should also be prepared to answer questions about machine learning concepts and tools. 6. Continue learning: Machine learning is a rapidly evolving field, so it’s important to stay up-to-date with the latest trends and technologies. You can do this by attending conferences, taking online courses, or participating in online communities. How Do Make Deepfakes? | How Generative Adversarial Networks (GANs) Work FAQs What do data labeling companies do? Data labeling companies provide services to help businesses and organizations label their data sets for machine learning purposes. These companies employ teams of data labelers who are trained to identify and categorize data, such as images, text, or audio, and label them with relevant tags or annotations. Data labeling companies work with a variety of industries, including healthcare, finance, and technology, to help them build and improve their machine learning models. They may also provide quality control services to ensure that the labeled data sets are accurate and consistent. Who are the largest data annotation companies? There are several large data annotation companies that provide services to businesses and organizations. Here are some of the largest ones: 1. Appen: Appen is a global leader in providing high-quality training data for machine learning and artificial intelligence. They offer a wide range of data annotation services, including image and video annotation, text annotation, and audio transcription. 2. Lionbridge: Lionbridge is a leading provider of translation, localization, and data annotation services. They offer a variety of data annotation services, including image and video annotation, text annotation, and speech recognition. 3. CloudFactory: CloudFactory is a data annotation company that specializes in providing high-quality training data for machine learning and artificial intelligence. They offer a variety of data annotation services, including image and video annotation, text annotation, and audio transcription. 4. Cogito: Cogito is a data annotation company that specializes in providing human-assisted artificial intelligence services. They offer a variety of data annotation services, including voice and speech annotation, sentiment analysis, and image and video annotation. 5. Scale AI: Scale AI is a data annotation company that provides high-quality training data for machine learning and artificial intelligence. They offer a variety of data annotation services, including image and video annotation, text annotation, and speech recognition. What is an example of data labeling? An example of data labeling is image classification. In this task, a data labeler is given a set of images and is asked to label each image with a relevant tag or category. For example, if the images are of animals, the labeler might be asked to label each image with the name of the animal in the picture, such as “dog”, “cat”, or “bird”. The labeled data set can then be used to
Amazon Mechanical Turk Reviews in 2023: Everything You Need to Know
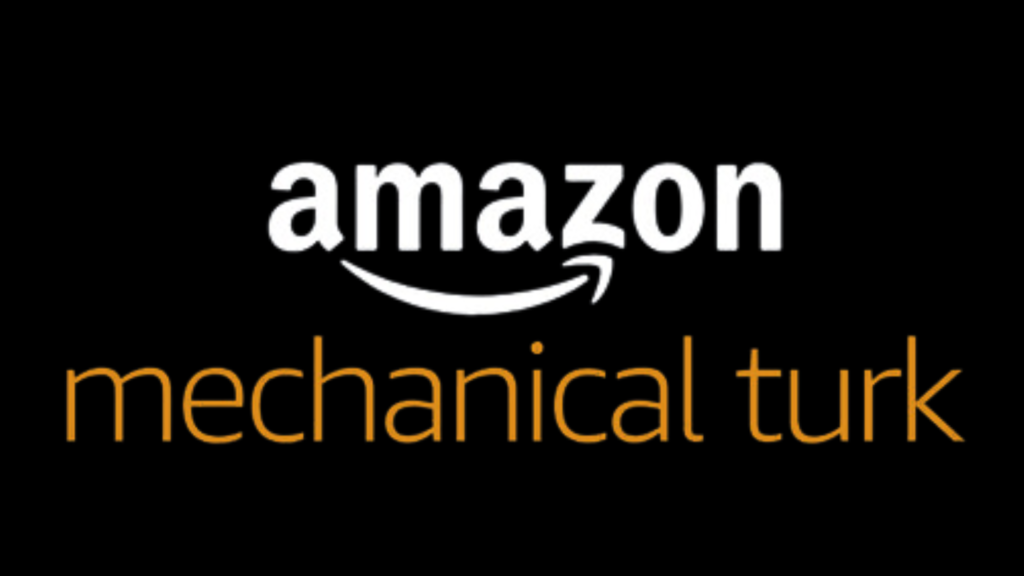
In this post, I’ll be sharing an in-depth review of MTurk. For those in the tech realm seeking clarity on leveraging crowdsourcing platforms for data-related tasks, this deep dive promises insightful revelations. So, prepare yourself to explore the intricacies of MTurk’s vast ecosystem with me. Earning Potential: Can you make good money with Amazon MTurk? Amazon Mechanical Turk allows workers to choose from thousands of tasks, with the pay rate for each task varying. Earnings from MTurk largely depend on factors commonly associated with a job. These include the type of tasks you choose, your efficiency, and the amount of time you’re willing to commit. Most workers spend a day or less per week working on Mechanical Turk and tend to complete 20-100 HITs during this time, generating a relatively low income stream of often less than $20 per week. Therefore, it seems that while it is possible to make some money on Amazon MTurk, it may not be a reliable source of primary income for most workers. The Process of Working on MTurk The process of working on MTurk begins with creating an account on the platform. After Amazon approves your registration, you can log in to the Amazon Mechanical Turk site. You can do this using your Amazon MTurk login credentials and browse through available HITs. Once you choose a HIT, complete it to the best of your ability, and submit it for approval. Once the requester approves your work, the payment will be credited to your Amazon Payments account. How Do Make Deepfakes? | How Generative Adversarial Networks (GANs) Work Understanding Amazon Mechanical Turk Jobs A requester can post a job, known as a Human Intelligence Task (HIT), on Mechanical Turk, which can be completed by workers in exchange for payment. HITs can be simple tasks, such as labeling images or transcribing audio, or more complex tasks, such as completing surveys or participating in experiments. Requesters can set the reward amount for each HIT, which can be as low as $0.01 and rarely exceeds $1. Workers can choose which HITs to complete based on the reward amount, the estimated time to complete the task, and other factors. Once a worker completes a HIT, the requester can approve or reject the work, and payment is made accordingly. Most workers spend a day or less per week working on Mechanical Turk and tend to complete 20-100 HITs during this time, generating a relatively low income stream of often less than $20 per week. However, there are some prolific workers who devote a significant amount of time and effort to completing thousands of HITs and claim to generate an income of more than $1000/month. Overall, understanding Amazon Mechanical Turk jobs involves understanding the process of posting HITs, completing tasks, and receiving payment, as well as the potential income and time commitment involved. Amazon Mechanical Turk Pay Amazon Mechanical Turk pays workers for completing Human Intelligence Tasks (HITs) posted by requesters. The payment for each HIT is set by the requester and can be as low as $0.01 and rarely exceeds $1 4. Once a worker completes a HIT, the requester can approve or reject the work, and payment is made accordingly. It is important to note that the income earned on Mechanical Turk can vary widely depending on the number and type of HITs completed, the payment amount for each HIT, and the time and effort invested by the worker. How Do Neural Networks work? | Learn Artificial Intelligence in Less than 10 Minutes References
Training Data vs Validation Data: What is the Difference
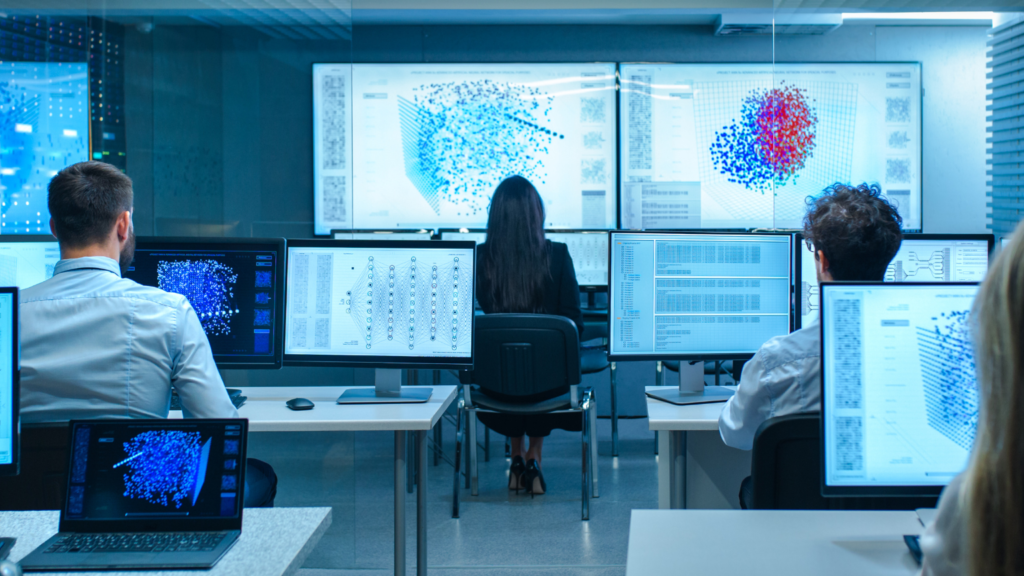
Machine learning algorithms are becoming increasingly popular in various fields, from finance to healthcare. However, one of the biggest challenges in machine learning is preventing overfitting, which occurs when a model is too complex and fits the training data too closely, resulting in poor performance on new, unseen data. In this article, we will explore the importance of training data and validation data in preventing overfitting, and discuss a new algorithm proposed by Andrew Y. Ng to overcome this issue. Training Data vs Validation Data In machine learning, the goal is to create a model that can accurately predict outcomes based on input data. To do this, we need to train the model on a set of data, known as the training data. The model learns from the training data and adjusts its parameters to minimize the error between the predicted outcomes and the actual outcomes. However, simply training the model on the training data is not enough. We also need to evaluate the model’s performance on new, unseen data, known as the validation data. This is important because the model may have learned to fit the training data too closely, resulting in poor performance on new data. By evaluating the model on the validation data, we can get an estimate of how well the model will perform on new data. CES 2023 Robotics Innovation Awards | Best New Robot Ventures Overfitting and Underfitting One of the biggest challenges in machine learning is finding the right balance between overfitting and underfitting. Overfitting occurs when the model is too complex and fits the training data too closely, resulting in poor performance on new data. Underfitting occurs when the model is too simple and fails to capture the underlying patterns in the data, resulting in poor performance on both the training data and new data. To prevent overfitting, we need to find the right balance between model complexity and model performance. This is where the validation data comes in. By evaluating the model on the validation data, we can get an estimate of how well the model will perform on new data. If the model performs well on the validation data, but poorly on the training data, it may be overfitting. If the model performs poorly on both the training data and the validation data, it may be underfitting.CES 2023 | When will robots take over the world? Preventing Overfitting with LOOCVCV In his paper, “Preventing Overfitting of Cross-Validation Data,” Andrew Y. Ng proposes a new algorithm, LOOCVCV, to prevent overfitting of cross-validation data. The algorithm involves selecting the hypothesis with the lowest cross-validation error, but with a twist. Instead of selecting the hypothesis with the lowest cross-validation error across all the training data, LOOCVCV selects the hypothesis with the lowest cross-validation error across all but one data point. This process is repeated for each data point, resulting in a set of hypotheses, each trained on all but one data point. The final hypothesis is then selected based on the average performance across all the hypotheses. This approach helps to prevent overfitting by ensuring that the model is not too closely fitted to any one data point. Experimental results show that LOOCVCV consistently outperforms selecting the hypothesis with the lowest cross-validation error. Limitations and Potential Drawbacks While LOOCVCV is a promising algorithm for preventing overfitting, it is not without its limitations and potential drawbacks. One limitation is that it may be computationally expensive, especially for large datasets. Another limitation is that it may not work well for datasets with a small number of data points, as there may not be enough data to train multiple hypotheses. Additionally, LOOCVCV may not be suitable for all types of machine learning problems. For example, it may not work well for problems where the input data is highly correlated, as removing one data point may not significantly change the model’s performance. Balancing Act: Train, Validation, Test Split Ratio The train, validation, and test split ratio is crucial. While there’s no hard and fast rule, a common split ratio is 70:15:15. That is, 70% of the data is used for training, and the remaining 30% is evenly split between validation and testing. However, these proportions might vary depending on the specifics of your dataset. Also, the proportions might change depending on the problem you’re trying to solve. The Bottom Line Preventing overfitting is a crucial step in machine learning, and requires finding the right balance between model complexity and model performance. By using both training data and validation data, we can evaluate the model’s performance on new, unseen data, and prevent overfitting. Andrew Y. Ng’s LOOCVCV algorithm is a promising approach to preventing the overfitting of cross-validation data and has shown consistent improvements over traditional methods. However, it is important to consider the limitations and potential drawbacks of the algorithm and to choose the approach that best fits the specific machine-learning problem at hand. References
Precision vs Accuracy Machine Learning: Understanding the Importance of Evaluation Metrics in Document Analysis
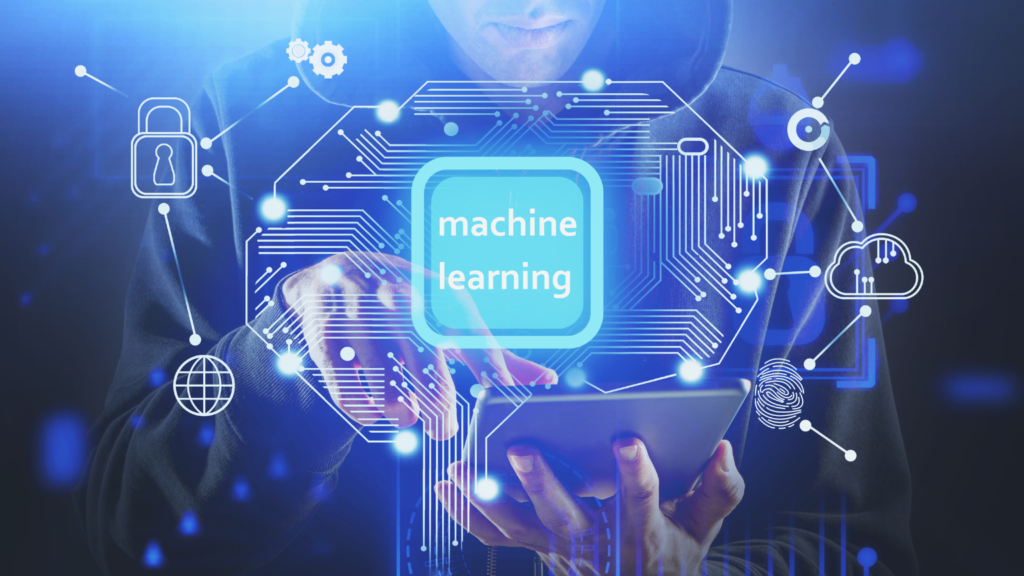
Machine learning has revolutionized the way we analyze and process data, especially in the field of document analysis. However, the effectiveness of machine learning algorithms depends on how well they are evaluated. In this article, we will explore the importance of evaluation metrics such as precision vs accuracy machine learning in document analysis. MIT Technology Review 35under35 | Jonas Cleveland | A Robotics Entrepreneur’s Motivation in Johnny5 The Process of Document Analysis Document analysis involves transforming printed documents into an electronic representation. This process involves several subtasks such as image processing, layout segmentation, structure recognition, and optical character recognition (OCR). One of the most important subtasks of document analysis is document categorization, which involves the automatic classification of documents into different types. This allows for workflow management, automatic routing or archiving of documents, and many other practical applications. To evaluate the effectiveness of document analysis systems, we need to use appropriate evaluation metrics. In document categorization, for example, we are interested in stating something about the performance of a particular algorithm. We are also interested in comparing different document categorization algorithms by their effectiveness. The effectiveness of text retrieved systems is usually given by the well-known IR standard measures recall and precision. Key Evaluation Metrics in Document Analysis Recall, precision, and accuracy are commonly used evaluation metrics in document analysis. Recall measures the proportion of relevant documents that are retrieved by a system, while precision measures the proportion of retrieved documents that are relevant. Accuracy measures the proportion of correct classifications made by a system. These metrics are essential in determining the effectiveness of document analysis systems. Statistical Reliability in Evaluation However, it is important to note that the experimental evaluation on relatively small test sets – as is very common in document analysis – has to be taken with extreme care from a statistical point of view. In fact, it is surprising how weak statements derived from such evaluations are. Therefore, it is important to use appropriate statistical methods to ensure that the results obtained are reliable. The effectiveness of machine learning algorithms in document analysis depends on how well they are evaluated. Evaluation metrics such as recall, precision, and accuracy are essential in determining the effectiveness of document analysis systems. However, it is important to use appropriate statistical methods to ensure that the results obtained are reliable. By understanding the importance of evaluation metrics, we can improve the effectiveness of machine learning algorithms in document analysis. Understanding Precision vs. Accuracy Precision and accuracy are two evaluation metrics that are often used interchangeably in machine learning. However, they have different meanings and are used in different contexts. Precision measures the proportion of true positives among all positive predictions, while accuracy measures the proportion of correct predictions among all predictions. In other words, precision measures how many of the predicted positive cases are actually positive, while accuracy measures how many of all cases are correctly predicted. Application Relevance In document analysis, precision and accuracy are both important metrics for evaluating the effectiveness of machine learning algorithms. Precision is particularly important in applications where false positives can have serious consequences, such as in medical diagnosis or fraud detection. In these cases, it is more important to avoid false positives than to maximize the number of true positives. For example, in a medical diagnosis system, a false positive could lead to unnecessary treatment or surgery, which can be harmful to the patient. On the other hand, accuracy is more important in applications where false negatives can have serious consequences, such as in security or safety-critical systems. In these cases, it is more important to avoid false negatives than to maximize the number of true positives. For example, in a security system, a false negative could allow unauthorized access to a secure area, which can be a serious security breach. In document analysis, precision and accuracy are often used together to evaluate the effectiveness of machine learning algorithms. For example, in document categorization, we may be interested in maximizing both precision and accuracy. This means that we want to maximize the number of relevant documents that are retrieved (high recall), while minimizing the number of irrelevant documents that are retrieved (high precision). At the same time, we want to maximize the number of correct classifications made by the system (high accuracy). Striking a Balance: Precision, Accuracy, and Their Trade-offs It is important to note that precision and accuracy are not always compatible metrics. In some cases, increasing precision may lead to a decrease in accuracy, and vice versa. For example, in a spam filter, increasing precision may require rejecting more emails as spam, which can lead to a decrease in accuracy if some legitimate emails are also rejected. Therefore, it is important to find a balance between precision and accuracy that is appropriate for the specific application. Conclusion In conclusion, precision and accuracy are important evaluation metrics in document analysis and machine learning in general. They measure different aspects of the effectiveness of machine learning algorithms and are used in different contexts. By understanding the differences between precision and accuracy and their appropriate use in different applications, we can improve the effectiveness of machine learning algorithms in document analysis and other fields. FAQs What’s the difference between precision and accuracy in machine learning? Accuracy in machine learning measures the ratio of correct predictions to the total number of predictions. Whereas precision measures the proportion of correctly predicted positive observations to the total predicted positives. In other words, accuracy gives an overview of the model’s performance, while precision provides a measure of the model’s reliability by predicting a positive class. What are the three types of precision? The three most common types of precision are: Precision as a classification metric: It measures the proportion of correctly predicted positive observations. Precision in numerical computations: It refers to the degree of numerical detail that a computational process can handle. Precision is a measure: It is a measurement of repeatability or reproducibility in fields like physics
Supervised Vs Unsupervised Machine Learning
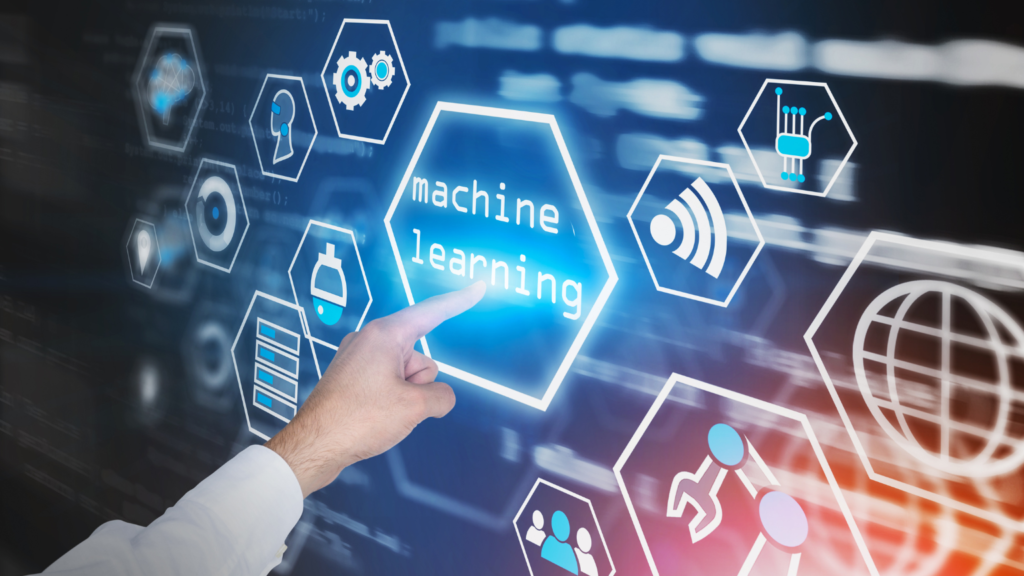
Machine learning is a rapidly growing field that has transformed our approach to data analysis. This involves algorithms and statistical models that derive insights without being explicitly programmed. Broadly speaking, machine learning models fall into two categories: supervised and unsupervised learning. In this guide, let’s delve into the distinctions between these two paradigms and how they’re leveraged in data mining. Supervised Vs Unsupervised Machine Learning Supervised Learning At its core, supervised learning involves the use of labeled data to train a model to make predictions or classifications. The labeled data consists of input variables (also known as features) and output variables (also known as labels or targets). The goal of supervised learning is to learn a mapping function that can predict the output variable given the input variables. Examples of supervised learning algorithms include neural networks, decision trees, and support vector machines. Neural Networks Type of supervised learning algorithm that is inspired by the structure and function of the human brain. They consist of multiple layers of interconnected nodes (also known as neurons) that process and transmit information. Neural networks are used for a wide range of applications such as image recognition, speech recognition, and natural language processing. Multi-Layer Perceptron (MLP) Multi-layer perceptron (MLP) is a type of neural network that consists of multiple layers of nodes. Each node in the input layer represents an input variable, and each node in the output layer represents an output variable. The nodes in the hidden layers perform computations on the input variables to generate the output variables. Decision Trees Decision trees are a type of supervised learning algorithm that is used for classification and regression tasks. They consist of a tree-like structure where each node represents a decision based on a feature of the input data. The leaves of the tree represent the output variables. Support Vector Machines (SVMs) Support vector machines (SVMs) are a type of supervised learning algorithm that is used for classification and regression tasks. They work by finding the hyperplane that maximally separates the data into different classes. SVMs are widely used in various applications such as image recognition, text classification, and bioinformatics.Tesla Robots Are Losing Direction | Robot SLAM Unsupervised Learning Unsupervised learning involves the use of unlabeled data to discover patterns and relationships in the data. The goal of unsupervised learning is to learn the underlying structure of the data without any prior knowledge of the output variable. Unsupervised learning is widely used in various applications such as anomaly detection, clustering, and dimensionality reduction. Clustering Clustering is a type of unsupervised learning algorithm that is used to group similar data points together. The goal of clustering is to partition the data into groups (also known as clusters) based on similarities. Clustering is widely used in various applications such as customer segmentation, image segmentation, and document clustering. K-Means A popular clustering algorithm that is used to partition the data into K clusters. The algorithm works by randomly selecting K centroids (also known as cluster centers) and assigning each data point to the nearest centroid. The centroids are then updated based on the mean of the data points assigned to each cluster. The algorithm iteratively repeats these steps until convergence. Self-Organizing Maps (SOMs) Self-organizing maps (SOMs) are a type of unsupervised learning algorithm that is used for dimensionality reduction and visualization. SOMs consist of a grid of nodes that are arranged in a two-dimensional space. Each node represents a weight vector that is updated based on the input data. The nodes that are close to each other in the grid represent similar data points. Ensembles in Machine Learning Type of machine learning technique that combines multiple models to improve the accuracy of predictions. Ensembles are widely used in various applications such as image recognition, speech recognition, and natural language processing. Bagging Type of ensemble technique that involves training multiple models on different subsets of the data and combining their predictions. Bagging is widely used in various applications such as random forests and gradient boosting. Boosting Type of ensemble technique that involves training multiple models sequentially, where each model is trained to correct the errors of the previous model. Boosting is widely used in various applications such as AdaBoost and XGBoost. Beyond Supervised and Unsupervised Learning Another vital branch of machine learning deserves mention: reinforcement learning. It’s a type where an agent learns to make decisions by taking actions in an environment to maximize some reward. The agent isn’t provided with correct input/output pairs or explicitly told to find hidden structures. Instead, it learns from rewards and punishments, distinguishing it from both supervised and unsupervised learning. Conclusion Supervised and unsupervised learning are two fundamental approaches to machine learning that have their unique advantages and disadvantages. Supervised learning is used when the output variable is known, and the goal is to learn a mapping function that can predict the output variable given the input variables. Unsupervised learning is used when the output variable is unknown, and the goal is to learn the underlying structure of the data. By understanding the differences between these two approaches, data scientists can choose the right approach for their specific data mining tasks and make informed decisions that lead to better insights and predictions. FAQs References
7 Best Image Classification Models You Should Know in 2023
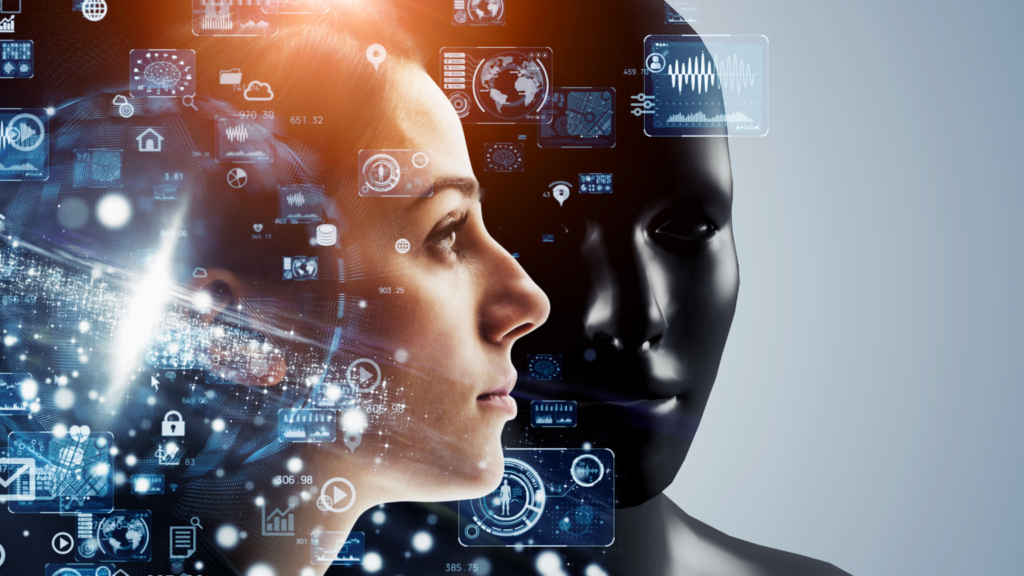
Image classification is a fundamental task in computer vision that involves assigning a label to an image based on its content. With the increasing availability of digital images, the need for accurate and efficient image classification models has become more important than ever. Convolutional neural networks (CNNs) have emerged as a powerful tool for image classification, achieving state-of-the-art performance on various datasets. In this article, we will explore the best image classification models based on a survey conducted by Wei Wang, Yujing Yang, Xin Wang, Weizheng Wang, and Ji Li. We will also compare various image classification methods and present experimental results of different models. Finally, we will highlight the latest innovations in network architecture for CNNs in image classification and discuss future research directions in the field. Also Check Will Innovation Save Us From Recession in 2023? | Investing in Generative AI Best Image Classification Models 1. Sparse coding: Sparse coding is a method of representing data in a high-dimensional space using a small number of basis functions. In image classification, sparse coding is used to learn a dictionary of basis functions that can be used to represent images. The method involves finding a sparse representation of an image by solving an optimization problem that minimizes the difference between the image and its representation in terms of the learned basis functions. Sparse coding has been used in image classification with some success, but it has been largely superseded by deep learning methods. 2. SIFT + FVs: Scale-Invariant Feature Transform (SIFT) is a method for detecting and describing local features in images. Fisher Vector (FV) is a method for encoding the distribution of local features in an image. SIFT + FVs is a popular method for image classification that involves extracting SIFT features from an image, encoding them using FVs, and using a classifier to predict the class of the image. SIFT + FVs has been used in several image classification competitions and has achieved state-of-the-art performance on some datasets. 3. AlexNet: AlexNet is a deep convolutional neural network that was developed by Alex Krizhevsky, Ilya Sutskever, and Geoffrey Hinton. It won the ImageNet Large Scale Visual Recognition Challenge (ILSVRC) in 2012 and was the first deep learning model to achieve state-of-the-art performance on the ImageNet dataset. AlexNet consists of five convolutional layers, followed by three fully connected layers. It uses the ReLU activation function and dropout regularization to prevent overfitting. AlexNet introduced several innovations that are now standard in deep learning, such as the use of GPUs for training and the use of data augmentation to increase the size of the training set. 4. VGGNet: VGGNet is a deep convolutional neural network that was developed by the Visual Geometry Group at the University of Oxford. It achieved second place in the ILSVRC 2014 competition and has been widely used in image classification tasks. VGGNet consists of 16 or 19 layers of convolutional and fully connected layers. It uses small 3×3 filters in all convolutional layers, which allows it to learn more complex features. VGGNet also introduced the use of batch normalization to improve the training of deep neural networks. 5. GoogLeNet/Inception: GoogLeNet, also known as Inception v1, is a deep convolutional neural network that was developed by researchers at Google. It won the ILSVRC 2014 competition and introduced the Inception module, which allows the network to learn features at multiple scales. The Inception module consists of parallel convolutional layers with different filter sizes, which are concatenated to form the output of the module. GoogLeNet also introduced the use of global average pooling, which reduces the number of parameters in the network and helps prevent overfitting. The network consists of 22 layers and has a relatively small number of parameters compared to other deep learning models. 6. ResNet: ResNet, short for Residual Network, is a deep convolutional neural network that was developed by researchers at Microsoft. It won the ILSVRC 2015 competition and introduced the concept of residual connections, which allow the network to learn residual functions instead of directly learning the underlying mapping. Residual connections help prevent the vanishing gradient problem that can occur in very deep neural networks. ResNet consists of many layers, with some versions having over 100 layers. It has been shown to achieve state-of-the-art performance on several image classification tasks. 7. DenseNet: DenseNet is a deep convolutional neural network that was developed by researchers at Facebook AI Research. It introduces the concept of dense connections, which connect each layer to every other layer in a feed-forward fashion. Dense connections allow the network to reuse features learned in previous layers and can help prevent overfitting. DenseNet consists of several dense blocks, each of which contains several convolutional layers and a transition layer that reduces the spatial dimensions of the output. DenseNet has achieved state-of-the-art performance on several image classification tasks and has been shown to be more parameter-efficient than other deep learning models. How to Survive the End of the World | Peter Zeihan Latest is Wrong References
Stable Diffusion Image To Image
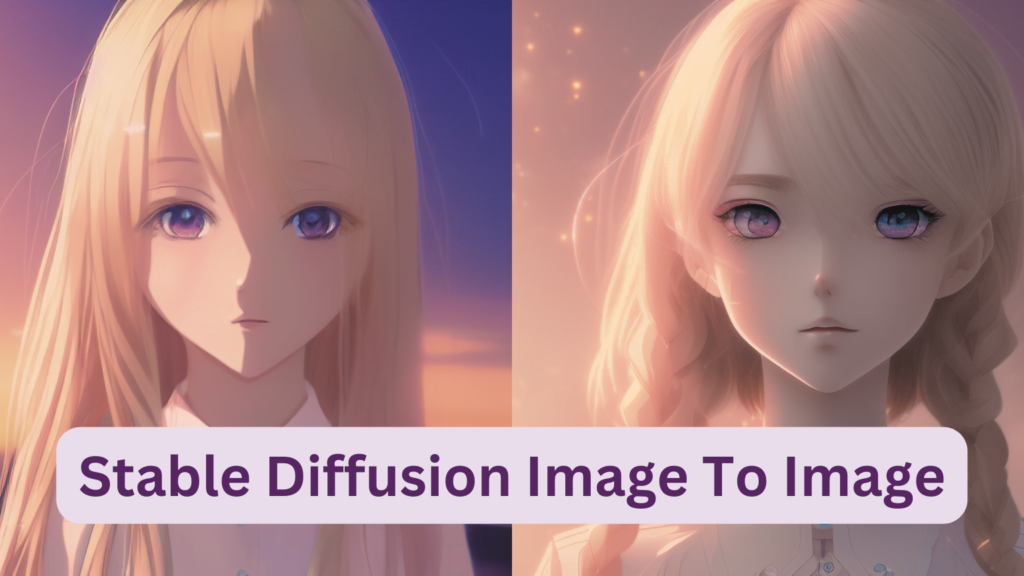
Stable Diffusion is a machine learning technique that generates high-quality images by predicting the next pixel in an image based on the previous pixels. This technique is based on the concept of diffusion, which is the process by which particles spread out over time. Stable Diffusion uses a diffusion model to predict the next pixel in an image based on the previous pixels. In this guide,I’ll explore the basics of Stable Diffusion and how to use it to create stunning images. Stable Diffusion Explained Stable diffusion is a technique used in generating high-quality images and audio. It is a state-of-the-art technique that uses a diffusion process to generate new creative output data. The process involves diffusing an image or audio signal over time, using a pre-trained model to generate new data. The technique is based on the concept of diffusion, which is a process of spreading out a signal over time. Stable diffusion is based on the concept of diffusion, which is a process of spreading out a signal over time. The technique involves diffusing an image or audio signal over time, using a pre-trained model to generate new data. The process is based on the idea of adding noise to the signal and then removing the noise to generate new data. The technique is designed to generate high-quality images and audio by using a diffusion process that is stable and robust. Will Innovation Save Us From Recession in 2023? | Investing in Generative AI Stable Diffusion Image To Image Diffusion models are the heart of Stable Diffusion. These models are trained on large datasets of images and learn to predict the next pixel in an image based on the previous pixels. There are several types of diffusion models, including autoregressive models, flow-based models, and diffusion-based models. Each type of model has its own strengths and weaknesses, and the choice of model depends on the specific application. Prompt Engineering Prompt engineering is the process of creating prompts that guide the generation of images. Prompts can be simple or complex, and they can be used to generate images of specific objects, scenes, or styles. The key to effective prompt engineering is to create prompts that are specific enough to guide the generation of high-quality images but not so specific that they limit the creativity of the model. Using Automatic1111’s Python Gradio Implementation Automatic1111’s Python Gradio implementation is a powerful tool for generating images with Stable Diffusion. This implementation provides a user-friendly interface for creating prompts and generating images. It also includes several options for optimizing the generation process, such as adjusting the temperature of the diffusion model and using dynamic lighting. Limitations and Challenges of Stable Diffusion While Stable Diffusion is a powerful tool for generating high-quality images, it is not without its limitations and challenges. Stable Diffusion Download and Online Availability The usage of Stable Diffusion is relatively straightforward as most models are open-source. You can easily download them from platforms like GitHub at your convenience. You can use these models in your local environment or deploy them in an online setting. Once installed, you can provide or feed the input image or text prompt to such a model. The chosen stable diffusion model will process it to produce the desired image output. Final Note Stable Diffusion is a powerful tool for generating high-quality images. By understanding the basics of diffusion models, you can create stunning images that are tailored to your specific needs. However, it is important to be aware of the limitations and challenges of Stable Diffusion. With these considerations in mind, Stable Diffusion can be a valuable tool for artists, designers, and researchers looking to generate high-quality images. References
Ranking the Best Image Annotation Tools of 2023
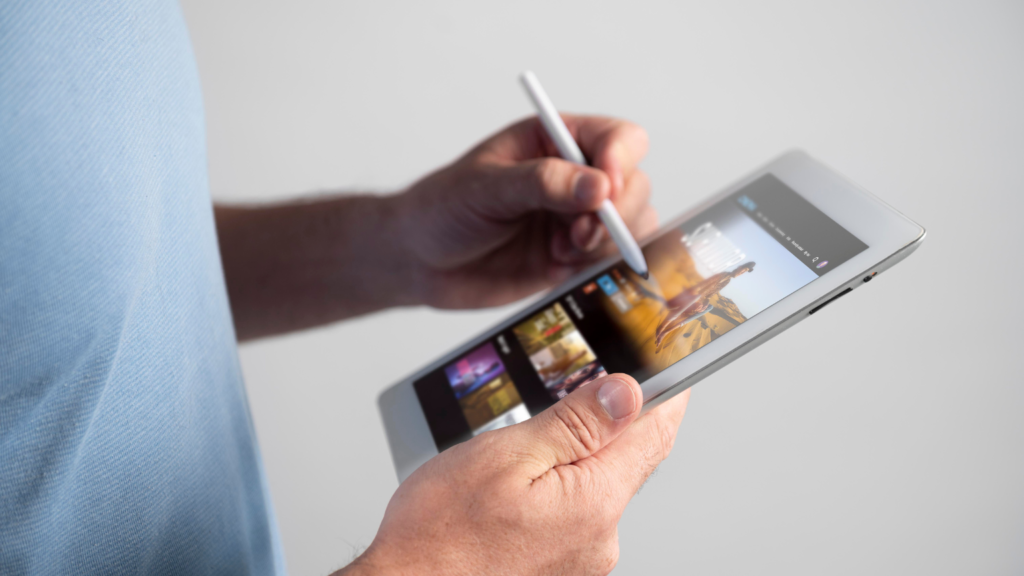
Quality data has always been the cornerstone of many breakthroughs in the AI world, especially in image processing. And at the heart of this data-centric evolution are image annotation tools, indispensable for refining our algorithms. Selecting the right tool can make the difference between a model that merely functions and one that excels. I’ve undertaken a comprehensive review of the top image annotation tools available in this article. For those entrenched in the AI trenches or anyone passionate about the mechanics behind the magic, this guide is your deep dive into the world of data labeling excellence. What is an Image Annotation Tool? An image annotation tool is a software application designed to manually mark and categorize objects within images. These tools enable humans to create meaningful data that machine learning models can learn from. Image annotation tools range from simple point-and-click interfaces to more complex applications. These complex annotation tools include features like segmentation, bounding boxes, and labeling and empowering a range of industries.AI Prompt Engineering | Easy AI Job. Top 10 Image Annotation Tools Annotation tools have numerous functionalities. From different data support to quality assurance, security, and integration capabilities, each tool is unique. We’ll delve into each based on these factors and keep you updated with the latest market entrants and features. 1. Encord Annotate: A Master in AI-Assisted Annotation Best for: Teams seeking advanced, AI-backed annotation and dataset management. Highlight: Multi-modal annotation support, including DICOM, NIfTI, and ultra-high-resolution. Cost: Initial free trial with subsequent user-based pricing. 2. Scale (formerly Scale AI): Expanding Horizons Beyond Image Annotation Best for: Emphasis on workforce management. Highlight: Broad annotation type support, from image to audio. Cost: Charged per image. 3. CVAT (Computer Vision Annotation Tool): Open-Source Excellence by Intel Best for: Academics and newbies to image annotation. Highlight: Varied annotation types and computer vision tasks support. Cost: Completely free. 4. Labelbox: A Powerhouse in Document and Text Annotations Best for: Teams prioritizing quick document and text annotations. Highlight: Seamless integration with third-party labeling services. Cost: Depends on various factors including data volume and usage. 5. Playment: End-to-End Managed Data Annotation Best for: A hassle-free, fully managed data annotation solution. Highlight: Massive global workforce of over a million annotators. Cost: Offered as an enterprise plan. 6. Appen: Pioneers in Data Labeling Best for: Comprehensive image data sourcing and labeling. Highlight: Broad annotation type support, including polygons and image segmentation. 7. Dataloop: A One-Stop Solution for All Annotation Needs Best for: Those seeking a versatile platform for varied data types. Highlight: User-friendly interface with support for image to video annotations. Cost: Free trial leading to an enterprise plan. 8. V7 Labs: Beyond Just Image Annotation Best for: Teams or students wanting a versatile annotation solution. Highlight: Comprehensive project management tools and real-time collaboration. Cost: Ranges from academic to pro options. 9. Hive: AI-Driven Content Moderation Solution Best for: Content moderation, especially for social media platforms. Highlight: Unique annotation applications, including logo detection. Cost: Enterprise model. 10. LabelMe: A Versatile Tool for Image Annotation Best for: Researchers and developers focusing on image segmentation and object detection. Highlight: Open-source platform with a user-friendly interface allowing polygonal region annotations in images. Cost: Free (Open-source software). Image Labeling Tool for Object Detection How Do Make Deepfakes? | How Generative Adversarial Networks (GANs) Work Conclusion: The Rise of Computer Vision Annotation Tools Image annotation tools play an indispensable role in the world of AI and machine learning. They are the unsung heroes behind successful AI applications, from autonomous vehicles to personalized shopping experiences. With the rise of these tools, the future of computer vision and AI is brimming with potential. There’s a wide array of data annotation tools to choose from, each adding value to the development of AI models. References
Unlocking Video Recognition with Open Source Technology
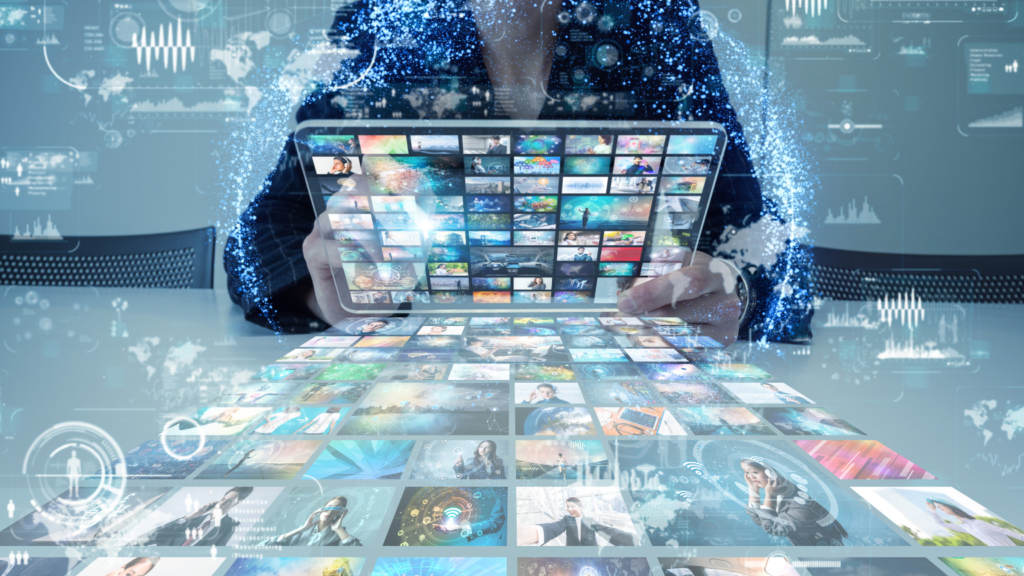
Video recognition, or video analytics, is a technology that automatically analyzes video feeds to recognize temporal and spatial events. It leverages machine learning algorithms to ‘understand’ the content of video data. This often includes identifying objects, people, text, and even specific actions or behavior. Now, let’s look at a related concept: image and video recognition. Both share the common goal of interpreting visual data, but they do so in different ways. Image recognition, also called computer vision, refers to a computer’s ability to identify and interpret individual images. Whereas video recognition extends this principle to motion-based data, analyzing sequences of images (i.e., video) over time. Top Five Remarkable Open-Source Object Detection Tools In this section, I highlight five outstanding open-source projects in Object Detection that you can leverage to advance your competencies in computer vision and image processing. 1. Imageai 2. AVOD – Aggregate View Object Detection: 3. Nudenet: 4. AI Basketball Analysis: 5. Vehicle Counting: Object Recognition Software: A Vital Component At the heart of many video recognition systems is object recognition software. Whereas object recognition is integral to understanding and interpreting visual data This technology identifies and classifies various objects within images or video frames. From recognizing a person’s face to identifying a type of vehicle, it can do wonders in terms of identification.. Conclusion Open-source video recognition software embodies the intersection of technology and creativity. The tools are vast, ranging from video action recognition systems to text-to-video AI, and their uses are expansive. By leveraging these resources, we can continue to harness the power of visual data and drive innovation. They can serve countless industries saving countless hours while producing new jobs. References