Demystifying Data Cleansing vs Data Cleaning: A Comparative Analysis
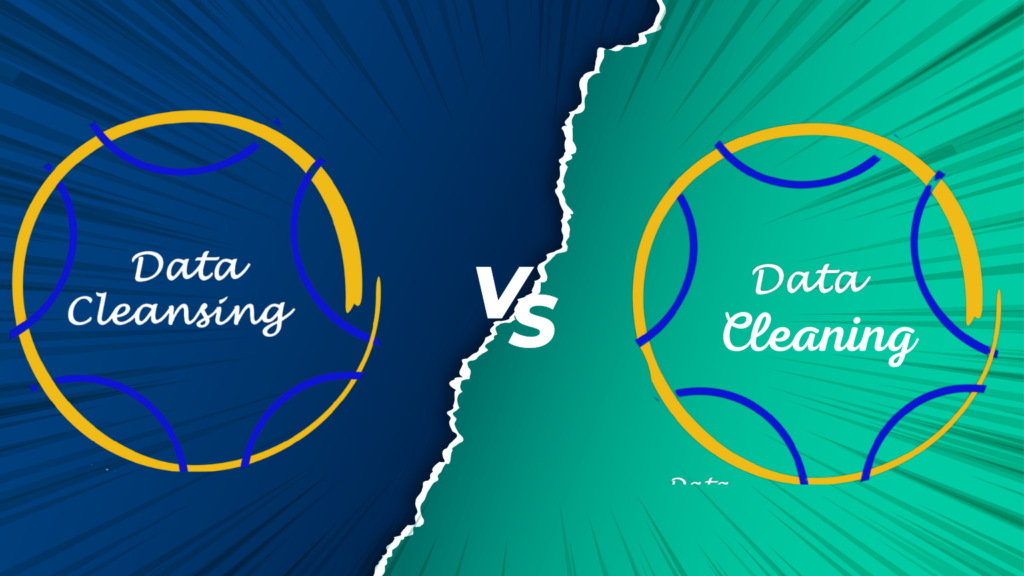
In the ever-evolving landscape of data science, terminologies can sometimes lead to confusion. Among such terms are “Data Cleansing” vs “Data Cleaning,” which are often used interchangeably. However, these two concepts differ in their scope and activities, and understanding their distinctions is crucial for data professionals. Data Cleaning, also known as data scrubbing, is a subset of the data cleansing process that involves detecting and rectifying errors or inconsistencies in data sets. On the other hand, Data Cleansing encompasses a more comprehensive approach, going beyond error detection to modify, replace, or delete dirty or coarse data, ultimately creating consistent and reliable data sets. This article aims to shed light on the unique aspects of data cleansing and data cleaning, exploring their processes, strengths, and weaknesses. By understanding these nuances, data professionals can make informed decisions on which approach best suits their specific needs, leading to more accurate and reliable data analysis and business insights. Best Data Labeling Company in 2023 Understanding the Terms: Data Cleaning and Data Cleansing Data Cleaning, often called data scrubbing, is a subset of the data cleansing process. It involves detecting and rectifying errors or inconsistencies from data sets, usually collected from disparate sources. It is a crucial step to increase data’s reliability and improve overall data quality. Conversely, Data Cleansing or data cleaning is a more comprehensive process. While it includes data cleaning as a component, it goes a step further. It involves not only finding and correcting errors but also modifying, replacing, or deleting dirty or coarse data to create consistent and reliable data sets. What’s the Difference Between Cleaning and Cleansing? The differentiation between data cleaning and data cleansing lies in the scope of activities performed. While both involve making data more accurate, useful, and reliable, data cleansing is more extensive. Cleaning refers to the identification and rectification of errors, while cleansing encompasses a broader set of operations. Cleansing refers to operations such as standardization, de-duplication, validation, and even enrichment with additional relevant data.Amazon Mechanical Turk Reviews in 2023: Everything You Need to Know Delving into the Data Cleansing Process The data cleansing process is a multi-stage operation that ensures data is accurate, complete, unique, and relevant. Here are some primary steps involved: Data Audit: The process begins with understanding the data’s current state, identifying the sources of dirty data, and defining rules for how data issues are handled. Workflow Specification: The next step involves establishing a workflow, outlining the sequence of data cleansing activities. Data Cleaning: This step involves actual cleaning, where errors are identified and rectified, and inconsistencies in data are resolved. Data Validation: Cleaned data is then validated, verifying if the cleaning has been performed accurately. It also ensures that the data now adheres to the defined rules and guidelines. Data Verification: The final step involves a manual verification of a subset of the data to ensure the automated cleansing process’s accuracy. Unraveling the Meanings: Cleaned Data vs Cleansed Data So, what is meant by cleaned data? Cleaned data is the output of the data cleaning process. It refers to data that has been examined and corrected for errors and inconsistencies. It is more accurate and reliable than the original data set, ensuring more accurate analytics and decision-making processes. Then what is meant by cleansed data? Well, cleansed data is the output of the data cleansing process. It goes beyond cleaned data by not only rectifying errors but also ensuring data standardization. Cleansing also represnt uniqueness, relevance, and even data enrichment. It is a higher-quality dataset, ready for further data analysis or processing. An Overview of Data Cleaning Steps Data cleaning steps, which form a part of the data cleansing process, mainly involve: Data Auditing: It involves identifying the anomalies and inaccuracies in the data and determining their sources. Data Correction: This step involves correcting identified errors, which might involve replacing inaccurate data with correct data or deleting irrelevant data. Data Verification: In this step, data is verified to ensure all inaccuracies have been addressed and corrected. Data Quality Assurance: The final step involves an additional layer of verification to ensure data quality before further data processing. Wrapping Up: Data Cleansing vs Data Cleaning Data cleaning and data cleansing might seem synonymous but they operate on different levels of data quality enhancement. Understanding the nuances can guide data professionals to choose the right approach for their specific needs. Remember, the key to successful data analysis and reliable business insights often lies in the robustness of the initial data cleaning or cleansing process. So, keep your data clean, or even better, cleansed!
Sharpen Your Skills with the Best Data Science Bootcamps
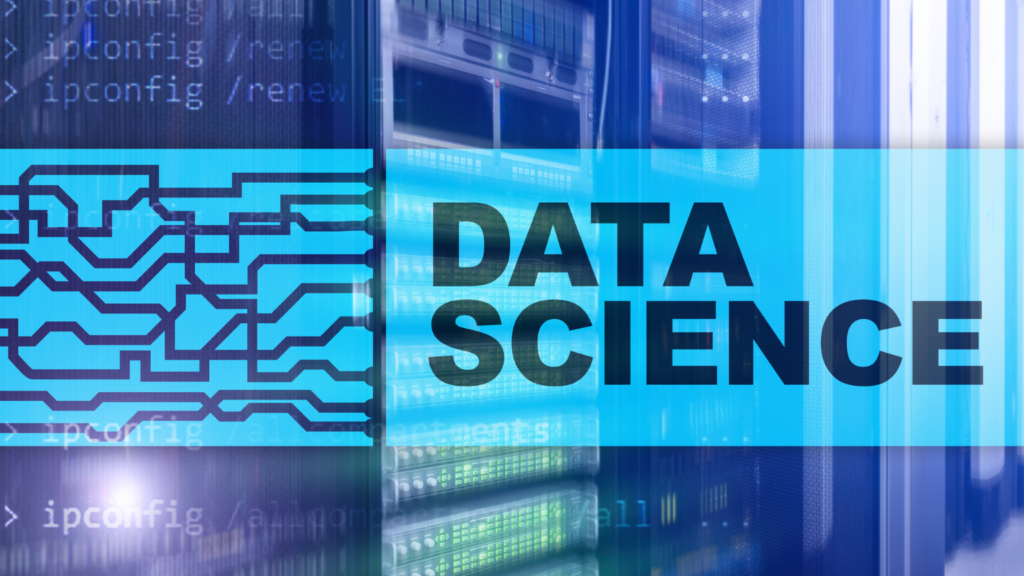
In a world that increasingly revolves around data, the demand for data scientists—experts who can turn that data into insights—is growing. As traditional education systems struggle to keep up with the fast-paced industry, alternative learning routes like data science boot camps have emerged. These boot camps offer intensive, focused training in data science in a short amount of time. But with a plethora of options, how can you find the best data science bootcamps? This article will guide you through the process, answering crucial questions and highlighting some top contenders. CES 2023 Robotics Innovation Awards | Best New Robot Ventures What is the Best Data Science Boot Camp? While the term “best” is relative and largely depends on individual needs, certain boot camps consistently rank high based on their comprehensive curriculum, skilled instructors, job placement success, and student reviews. One such boot camp is the Springboard Data Science Bootcamp. This program stands out with its job placement guarantee and one-on-one mentoring from industry professionals. Springboard’s rigorous curriculum includes both theoretical instruction and practical projects, ensuring students acquire the necessary skills for a career in data science. Is a Bootcamp Enough for Data Science? This question often arises among individuals considering alternative learning paths. Can a boot camp, typically lasting only a few weeks or months, equip you with the skills required for a data science career? The answer is yes and no. Boot camps can provide an intensive, concentrated introduction to data science. They cover key concepts and skills, offer practical projects, and sometimes even provide career services like networking and job placement assistance. For individuals with some background in mathematics or coding, a boot camp can be an effective way to quickly transition into data science. However, data science is a vast field that continues to evolve. Even after a boot camp, you’ll likely need to continue learning and expanding your skills, either on the job or through additional courses. Which Training Institute is Best for Data Science? In addition to boot camps, several reputable training institutes offer comprehensive data science courses. Institutions like General Assembly, DataCamp, and Coursera are often lauded for their extensive online data science courses. These platforms offer flexibility and a wide array of courses catering to different skill levels—from beginners to experienced professionals. Free and In-Person Data Science Boot Camps While many boot camps come with hefty price tags, there are also free options available. These free courses, like the one offered by Dataquest, may not provide as much personalized attention or career services, but they still cover important data science concepts. For individuals who prefer in-person learning, options are available too. Schools like General Assembly and Metis offer in-person data science boot camps in various cities across the U.S. However, due to COVID-19, many of these programs have temporarily shifted online. Data Science Boot Camp Near Me When seeking a data science boot camp, location is an important factor for those preferring in-person learning. Boot camps are available in major cities across the globe, from New York to London to Singapore. Using simple online searches or platforms like CourseReport or Switchup, you can find boot camps near your location. CES 2023 | When will robots take over the world? Data Science Bootcamp Cost The cost of data science boot camps varies greatly, ranging from free to over $10,000. Factors influencing cost include the length of the program, whether it’s in-person or online, and the resources provided, such as career services or mentorship. While cost is an important consideration, it’s crucial to evaluate the return on investment. Many high-quality boot camps offer job placement guarantees or tuition refunds, making them a worthy investment. In conclusion, finding the best data science boot camp involves considering a variety of factors, including curriculum depth, format, cost, and your individual career goals. While a boot camp can provide a robust foundation, remember that in the ever-evolving field of data science, learning is a lifelong journey. Whether you choose a free online course, an intensive in-person boot camp, or a comprehensive program at a training institute, the best boot camp is the one that suits your learning style, meets your needs, and empowers you to unlock the potential of data. References
Breaking Language Barriers: The Best NLP Projects in AI
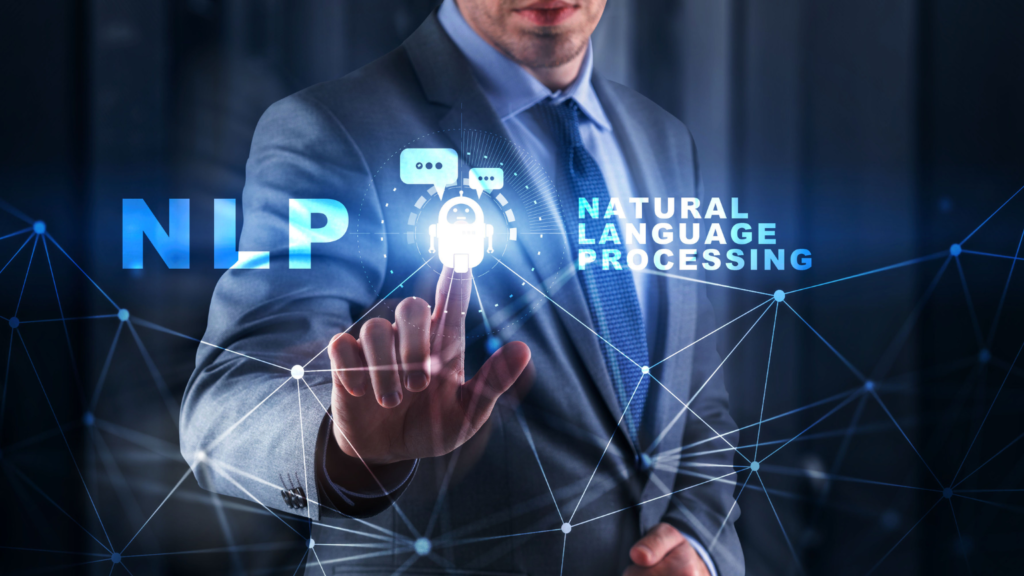
NLP has become increasingly popular in recent years, with many companies and organizations using it to improve their customer service, automate tasks, and gain insights from large amounts of text data. In this article, we will explore some of the best NLP projects in Al. I’ll discuss how these projects can be used in various industries and how they can help companies stay ahead of the competition. Whether you are a business owner, a data scientist, or simply interested in the latest developments in AI, this article will provide you with valuable insights into the world of NLP and its practical applications. The Best NLP Projects in AI Natural Language Processing (NLP) is a subfield of Artificial Intelligence (AI) that focuses on the interaction between computers and humans using natural language. 1. Sentiment Analysis Sentiment analysis is a popular NLP project that involves analyzing text data to determine the sentiment or emotion behind it. This can be useful for companies that want to understand how their customers feel about their products or services. Sentiment analysis can be used in the healthcare industry to analyze patient feedback and improve the quality of care. It can be used in social media monitoring to track brand reputation and customer satisfaction. 2. Chatbots Chatbots are another popular NLP project that involves creating a computer program that can simulate human conversation. Chatbots can be used for a variety of purposes, such as customer service, sales, and marketing. Chatbots can be used in the banking industry to provide customers with personalized financial advice and assistance. It can be used in the hospitality industry to provide guests with information about their stay and answer any questions they may have. 3. Text Summarization Text summarization is an NLP project that involves creating a summary of a longer piece of text. This can be useful for companies that want to quickly analyze large amounts of text data. Text summarization can be used in the legal industry to quickly analyze legal documents and identify key information. It can be used in the news industry to quickly summarize news articles and provide readers with a brief overview of the story. 4. Named Entity Recognition Named Entity Recognition (NER) is an NLP project that involves identifying and classifying named entities in text data, such as people, organizations, and locations. NER can be useful for companies that want to extract information from large amounts of text data. NER can be used in the healthcare industry to extract information from patient records and identify patterns in patient data. It can be used in the finance industry to extract information from financial reports and identify key financial indicators. 5. Machine Translation Machine translation is an NLP project that involves translating text from one language to another using a computer program. Machine translation can be useful for companies that operate in multiple countries and need to communicate with customers and employees in different languages. Machine translation can be used in the legal industry to translate legal documents and contracts into different languages. Machine translation can be used in the tourism industry to translate tourist travel guides and information. 6. Speech Recognition Speech recognition is an NLP project that involves converting spoken language into text using a computer program. Speech recognition can be useful for companies that want to automate tasks and improve accessibility for people with disabilities. Speech recognition can be used in the healthcare industry to transcribe patient conversations and improve the accuracy of medical records. It can be used in the automotive industry to create voice-activated controls for cars. Also check Data Labeling Company Open Source NLP Projects: Collaboration on GitHub GitHub is a treasure trove of open-source NLP projects, providing a platform for collaboration and knowledge-sharing. You can explore repositories like nltk, spaCy, and Hugging Face Transformers. These offer pre-trained models, code examples, and resources to kick-start your NLP project. Such projects facilitate accelerated development and foster a vibrant NLP community. Conclusion In conclusion, NLP is a rapidly growing field in AI that has many practical applications for businesses and organizations. From sentiment analysis to speech recognition, there are many NLP projects that can help companies automate tasks, gain insights from text data, and improve customer service. By leveraging the power of NLP, companies can stay ahead of the competition and provide better experiences for their customers and employees. References
How To Make Money On Amazon Mechanical Turk: A Comprehensive Guide
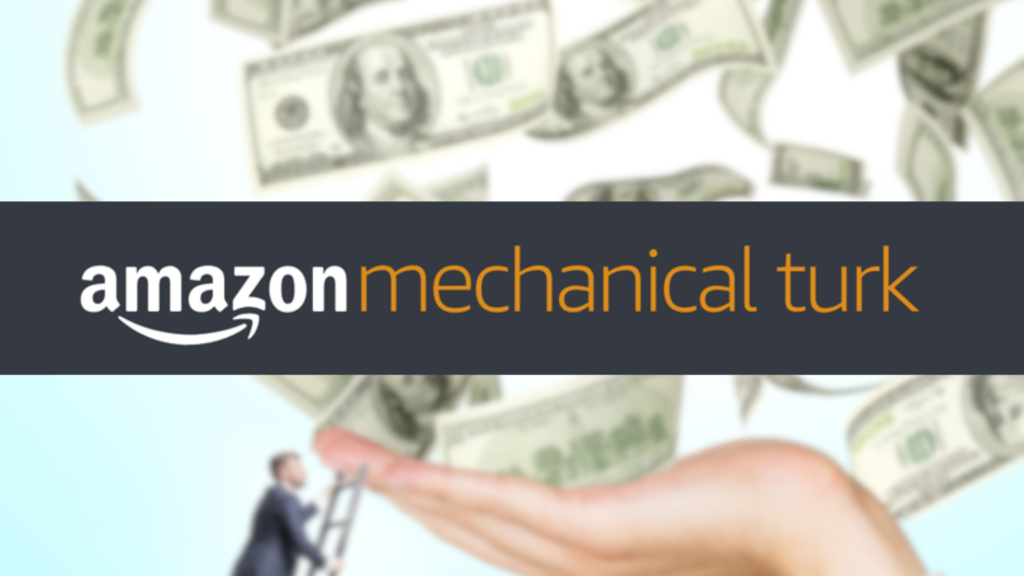
Amazon Mechanical Turk (MTurk), a crowdsourcing marketplace, offers a unique way to make money online. MTurk connects businesses and individuals, known as requesters, with a diverse, on-demand workforce, aptly named ‘Turkers’. Tasks ranging from data validation and research to content moderation are posted on the platform. MTurk offers a unique avenue for individuals to monetize their skills. Navigating its waters, however, requires a blend of strategic insight and technical know-how. Understanding Amazon Mechanical Turk MTurk provides a platform for completing micro-tasks known as Human Intelligence Tasks (HITs). These tasks, often ones that require a human touch, vary significantly in nature and complexity. Some examples of HITs include image or text classification, content creation, completing surveys, and much more. Your earnings on MTurk will depend on the type of HITs you choose and the efficiency with which you complete them.Amazon Mechanical Turk Reviews Can You Really Make Money on Mechanical Turk? The short answer is yes. However, as with any online platform, there are nuances to consider. On MTurk, you can earn money by completing HITs, but the amount you can earn varies greatly. It all depends on the complexity of tasks, your experience, and duration of the tasks. The question “How much money do you make as an Amazon Mechanical Turk?” does not have a one-size-fits-all answer. While some Turkers report earning a few hundred dollars a month, others might find their earnings to be lower. The key to maximizing your earnings is to be selective about the tasks you choose and to work efficiently. Making the Most of Your Time on MTurk A strategic approach is vital when aiming to make good money with Amazon MTurk. Some HITs that pay just a few cents might only take a few minutes, making them more lucrative than they initially seem. So, focus on tasks that align with your skill set and pay a fair wage for the time required. It can be beneficial to specialize in certain types of tasks. For example, if you’re fast at typing, Amazon Mechanical Turk data entry tasks might be a good fit for you. However, you will need a good eye and must pay good attention to details.Training Data vs Validation Data Daily Earnings on Amazon Mechanical Turk The question “How much can you make on Amazon Mechanical Turk a day?” is dependent on several variables. These include the number of hours you can dedicate, the type of HITs available, and the speed at which you can accurately complete those HITs. As you gain experience and get better at choosing and completing HITs, your daily earnings may increase. However, it is important to maintain realistic expectations about potential earnings. Consider MTurk as a way to supplement your income rather than a primary income source. Amazon Mechanical Turk Pay The pay rate for each task varies depending on the requester and the nature of the task. Amazon Mechanical Turk pay rates can range from a few cents to several dollars per task. Some requesters also offer bonuses for high-quality work or for completing a large number of HITs. While it might be tempting to focus on high-paying HITs, don’t overlook the potential of smaller tasks. Many smaller tasks can be completed quickly and can be lucrative in the long run. They require less time which means you could earn a substantial amount when these earnings are accumulated. Amazon Mechanical Turk: A Global Perspective While MTurk provides a platform for earning money to a global workforce, its availability varies by country. As of the last update in September 2021, MTurk was only available to workers in selected countries. Amazon Mechanical Turk can be a viable platform for making money online, especially for those who are multitaskers and enjoy flexibility. To maximize your earnings, choose tasks that align with your skills, work efficiently, and dedicate a consistent amount of time. While your earnings on MTurk may not replace a full-time income, it can provide a useful supplemental income. However, ensure that you’re aware of the platform’s availability in your country before getting started. With patience and strategy, you can make the most out of your time on Amazon Mechanical Turk. FAQs References