In the ever-evolving landscape of data science, terminologies can sometimes lead to confusion. Among such terms are “Data Cleansing” vs “Data Cleaning,” which are often used interchangeably. However, these two concepts differ in their scope and activities, and understanding their distinctions is crucial for data professionals. Data Cleaning, also known as data scrubbing, is a subset of the data cleansing process that involves detecting and rectifying errors or inconsistencies in data sets. On the other hand, Data Cleansing encompasses a more comprehensive approach, going beyond error detection to modify, replace, or delete dirty or coarse data, ultimately creating consistent and reliable data sets.
This article aims to shed light on the unique aspects of data cleansing and data cleaning, exploring their processes, strengths, and weaknesses. By understanding these nuances, data professionals can make informed decisions on which approach best suits their specific needs, leading to more accurate and reliable data analysis and business insights.
Best Data Labeling Company in 2023
Understanding the Terms: Data Cleaning and Data Cleansing
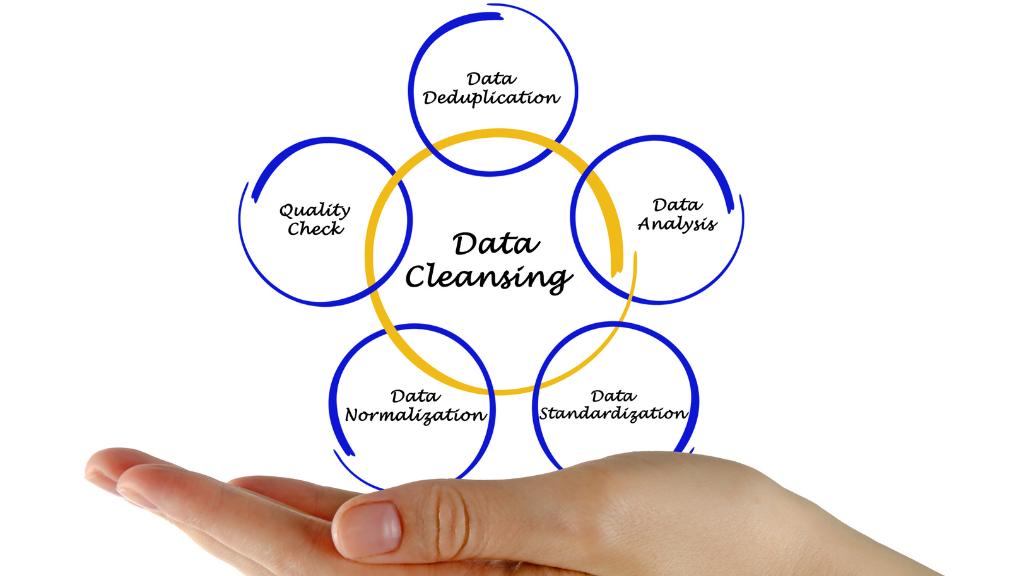
Data Cleaning, often called data scrubbing, is a subset of the data cleansing process. It involves detecting and rectifying errors or inconsistencies from data sets, usually collected from disparate sources. It is a crucial step to increase data’s reliability and improve overall data quality.
Conversely, Data Cleansing or data cleaning is a more comprehensive process. While it includes data cleaning as a component, it goes a step further. It involves not only finding and correcting errors but also modifying, replacing, or deleting dirty or coarse data to create consistent and reliable data sets.
What’s the Difference Between Cleaning and Cleansing?
The differentiation between data cleaning and data cleansing lies in the scope of activities performed. While both involve making data more accurate, useful, and reliable, data cleansing is more extensive. Cleaning refers to the identification and rectification of errors, while cleansing encompasses a broader set of operations. Cleansing refers to operations such as standardization, de-duplication, validation, and even enrichment with additional relevant data.
Amazon Mechanical Turk Reviews in 2023: Everything You Need to Know
Delving into the Data Cleansing Process
The data cleansing process is a multi-stage operation that ensures data is accurate, complete, unique, and relevant. Here are some primary steps involved:
Data Audit: The process begins with understanding the data’s current state, identifying the sources of dirty data, and defining rules for how data issues are handled.
Workflow Specification: The next step involves establishing a workflow, outlining the sequence of data cleansing activities.
Data Cleaning: This step involves actual cleaning, where errors are identified and rectified, and inconsistencies in data are resolved.
Data Validation: Cleaned data is then validated, verifying if the cleaning has been performed accurately. It also ensures that the data now adheres to the defined rules and guidelines.
Data Verification: The final step involves a manual verification of a subset of the data to ensure the automated cleansing process’s accuracy.
Unraveling the Meanings: Cleaned Data vs Cleansed Data
So, what is meant by cleaned data?
Cleaned data is the output of the data cleaning process. It refers to data that has been examined and corrected for errors and inconsistencies. It is more accurate and reliable than the original data set, ensuring more accurate analytics and decision-making processes.
Then what is meant by cleansed data?
Well, cleansed data is the output of the data cleansing process. It goes beyond cleaned data by not only rectifying errors but also ensuring data standardization. Cleansing also represnt uniqueness, relevance, and even data enrichment. It is a higher-quality dataset, ready for further data analysis or processing.
An Overview of Data Cleaning Steps
Data cleaning steps, which form a part of the data cleansing process, mainly involve:
Data Auditing: It involves identifying the anomalies and inaccuracies in the data and determining their sources.
Data Correction: This step involves correcting identified errors, which might involve replacing inaccurate data with correct data or deleting irrelevant data.
Data Verification: In this step, data is verified to ensure all inaccuracies have been addressed and corrected.
Data Quality Assurance: The final step involves an additional layer of verification to ensure data quality before further data processing.
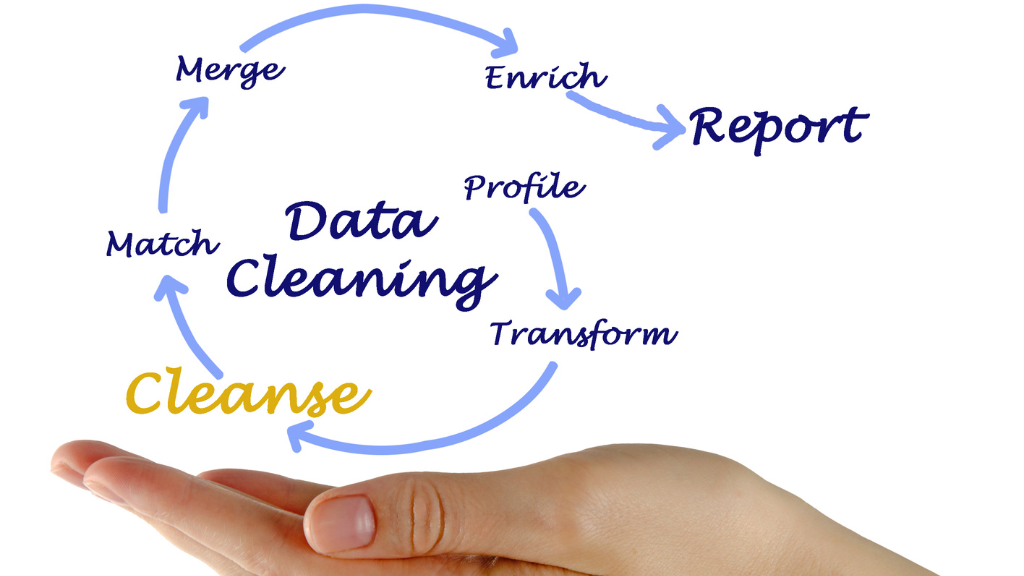
Wrapping Up: Data Cleansing vs Data Cleaning
Data cleaning and data cleansing might seem synonymous but they operate on different levels of data quality enhancement. Understanding the nuances can guide data professionals to choose the right approach for their specific needs. Remember, the key to successful data analysis and reliable business insights often lies in the robustness of the initial data cleaning or cleansing process. So, keep your data clean, or even better, cleansed!
What is the difference between data cleaning and data cleansing?
Data cleaning is a part of data cleansing and involves the detection and rectification of errors and inconsistencies in datasets. Data cleansing, however, is a more comprehensive process that, besides cleaning, includes standardization, validation, de-duplication, and sometimes enrichment of the data.
Is data cleaning necessary before data analysis?
Is data cleaning necessary before data analysis?
What does the data cleansing process entail?
The data cleansing process includes several steps like data auditing, workflow specification, data cleaning, data validation, and data verification. It ensures the data is not just error-free, but also standardized, unique, and relevant.
What is meant by cleaned data?
Cleaned data is data that has been processed through the data cleaning stage. It has been checked and corrected for errors and inconsistencies, making it more accurate and reliable than the original unprocessed data.
What is meant by cleansed data?
Cleansed data refers to data that has gone through the comprehensive data cleansing process. It is not only free from errors and inconsistencies but also standardized, validated, and often enriched, making it ready for further data processing or analysis.
How often should data cleansing be performed?
The frequency of data cleansing depends on the data’s nature and the use case. However, it’s generally a good practice to cleanse data whenever new data is added to the dataset or when the data is used for a new purpose.
Can data cleansing improve data analysis outcomes?
Absolutely. Data cleansing improves the quality of the data, reducing the likelihood of errors in data analysis. This leads to more accurate results and better decision-making.
Can data cleaning and cleansing be automated?
Yes, several aspects of data cleaning and cleansing can be automated using specific tools and software. However, manual oversight and verification are still important to ensure the quality and accuracy of the cleaned and cleansed data.