Text annotation is a crucial task in natural language processing (NLP) that involves labeling text data for training and validation. However, manual annotation is a time-consuming and expensive process that can suffer from inconsistencies and errors due to human limitations. To address these challenges, researchers have recently explored the potential of generative large language models (LLMs) to replace human annotators. In this article, we will explore the best AI annotation tools available and how they are revolutionizing the field.
Best AI Annotation Tools
AI annotation tools are software applications that use machine learning algorithms to help annotate data. These tools can be used to label images, videos, audio, and text data. They can also be used to generate training data for machine learning models. AI annotation tools can help improve the efficiency and quality of data labeling, making it easier to generate large datasets for AI models.
There are many AI annotation tools currently available, but some stand out because of their ability to improve the efficiency and quality of data labeling. Here are some of the best AI annotation tools.
Labelbox
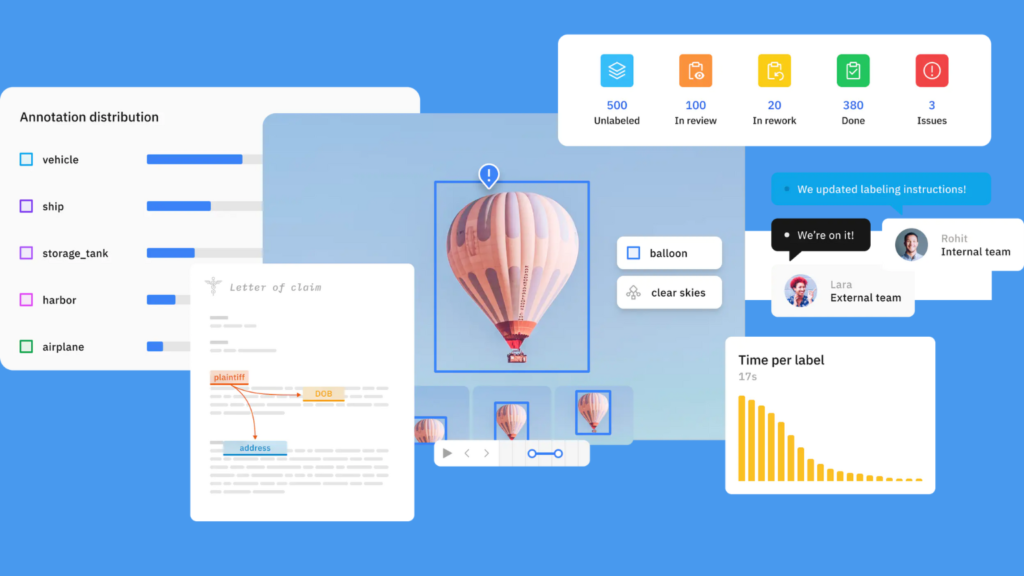
Labelbox is a data labeling platform that allows users to create, manage, and annotate datasets for machine learning models. It provides a user-friendly interface for annotators to label images, videos, and text data. Labelbox also offers features such as quality control, collaboration, and automation to help improve the efficiency and quality of data labeling.
OpenAI’s GPT-4 API
OpenAI’s GPT-4 API is one of the most popular AI annotation tools available. It is a generative LLM that can generate high-quality labels for a variety of NLP tasks. GPT-4 is trained on large amounts of text data and can be fine-tuned on specific tasks to improve its performance. It is also relatively affordable, with a cost of around $20 USD to annotate a dataset with 1,000 text samples.
Hugging Face’s Transformers
Hugging Face’s Transformers is another popular AI annotation tool that is built on top of PyTorch and TensorFlow. It offers a wide range of pre-trained models for various NLP tasks, including text classification, named entity recognition, and question answering. Transformers also allows users to fine-tune pre-trained models on their own datasets to improve their performance.
Amazon SageMaker Ground Truth
Amazon SageMaker Ground Truth is a fully managed data labeling service that uses machine learning to reduce the time and cost of manual annotation. It offers a variety of annotation workflows, including image classification, text classification, and named entity recognition. SageMaker Ground Truth also allows users to create custom annotation workflows and integrate them with their own machine-learning models.
Prodigy
Prodigy is a data annotation tool that is designed for machine learning practitioners. It offers a variety of annotation workflows, including text classification, named entity recognition, and image classification. Prodigy also allows users to create custom annotation workflows and to integrate with their own machine learning models. It is a paid tool, with a cost of $390 USD for a single user license.
Labelbox Labelbox is a data annotation platform that offers a variety of annotation workflows, including text classification, named entity recognition, and image classification. It also offers collaboration features that allow multiple users to work on the same dataset. Labelbox is a paid tool, with a cost of $ 200 USD per month for a single-user license.
Snorkel
Snorkel is a data labeling and management platform that uses weak supervision to reduce the time and cost of manual annotation. It allows users to create labeling functions that generate labels based on patterns in the data, rather than relying on human annotators. Snorkel also offers a variety of annotation workflows, including text classification, named entity recognition, and image classification.
Google Cloud AutoML
Google Cloud AutoML is a suite of machine learning tools that includes a data labeling service. It offers a variety of annotation workflows, including text classification, named entity recognition, and image classification. AutoML also allows users to create custom machine learning models using their labeled data.
SuperAnnotate
SuperAnnotate is a data annotation platform that uses AI to help improve the efficiency and quality of data labeling. It provides a user-friendly interface for annotators to label images, videos, and text data. SuperAnnotate also offers features such as quality control, collaboration, and automation to help improve the efficiency and quality of data labeling. It uses AI to suggest annotations to annotators, which can help speed up the labeling process.
Dataturks
Dataturks is a data annotation platform that allows users to create, manage, and annotate datasets for machine learning models. It provides a user-friendly interface for annotators to label images, videos, and text data. Dataturks also offers features such as quality control, collaboration, and automation to help improve the efficiency and quality of data labeling. It uses AI to suggest annotations to annotators, which can help speed up the labeling process.
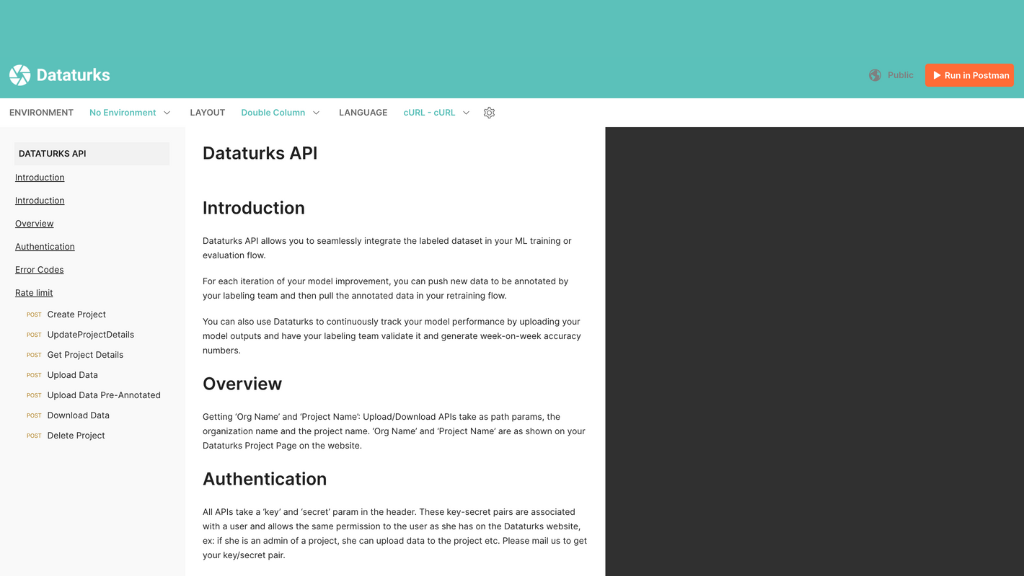
Tpu vs Gpu: The Giants of Computational Power
The Benefits of AI Annotation
AI annotation offers several benefits over manual annotation. First, it is faster and more cost-effective, as LLMs can annotate large amounts of text data in a fraction of the time it would take human annotators. Second, it can improve the quality of labeled text data by reducing inconsistencies and errors caused by human limitations. Third, it can be used to annotate text data in languages that may not have enough human annotators available.
The Future of AI Annotation
AI annotation is still a relatively new field, and there is much research to be done to improve the performance of LLMs in text annotation. One area of research is developing LLMs that can generate labels for more complex NLP tasks, such as sentiment analysis and named entity recognition. Another area of research is developing LLMs that can generate labels for non-textual data, such as images and audio.
Conclusion
AI annotation tools are becoming increasingly important in various fields, including biology, computer vision, and natural language processing. They can help improve the efficiency and quality of data labeling, making it easier to generate large datasets for AI models.