In the vast world of big data, we are faced with many buzzwords. Right now, the dialogue has been dominated by two intriguing concepts: Data Lakes and Lake Houses. While both harbor vast reservoirs of data, their architectural philosophies and operational nuances differentiate them substantially. As the amount of data we generate skyrockets, understanding these intricacies becomes essential for optimal data strategy formulation.
This blog post is designed as a deep dive into the world of Data Lakes and Lake Houses. We’ll try identifying their differences as well as the advantages that each offers to your business. By the end of our exploration, you should be able to answer the burning question: Data Lake vs Lakehouse: Which one is right for your organization?
Electric Car Efficiency vs Gas: A Comprehensive Comparison
Understanding the Fundamentals: Data Lake
Firstly, let’s dive into the concept of a Data Lake. Think of a Data Lake as a vast reservoir that holds a large amount of raw, unstructured data in its native format. Data Lakes are designed to store data at any scale, be it small or “big data”. These can accommodate data from various sources including social media feeds, IoT devices, transactional data, and more.
Data Lakes are particularly useful for data scientists and analysts. They provide an unhindered view of data, allowing these professionals to conduct deep analytics and machine learning operations. As the data is unprocessed and raw, users can query and analyze it in myriad ways. Thus, it provides fresh insights that a structured data environment may not readily provide.
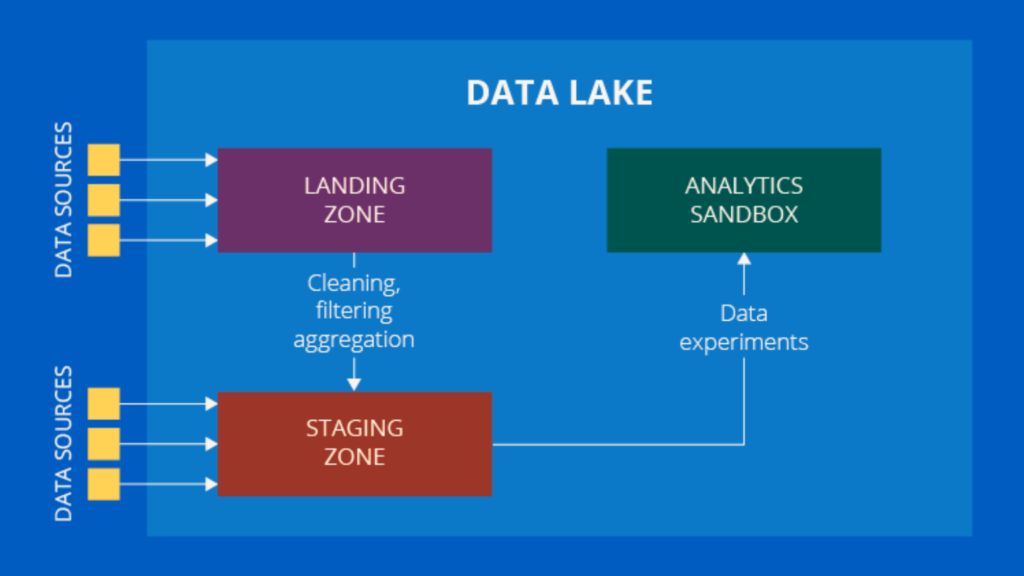
Introducing Lakehouse
While Data Lakes provide flexible data storage and retrieval, the idea of a Lakehouse aims to marry the best of Data Lakes and Data Warehouses. A Lakehouse is a new, hybrid data management paradigm It combines the storage flexibility of Data Lakes with the reliable data structuring of Data Warehouses.
In essence, a Lakehouse allows businesses to store both structured and unstructured data. It permits an extensive range of analytics from dashboards and reports to real-time analytics and machine learning. A Lakehouse maintains a unified, open platform that handles all data types and all workloads. Thus, eliminating the need for different architectures for disparate data tasks.
This streamlined, unified approach sets the Lakehouse apart in the data management landscape.
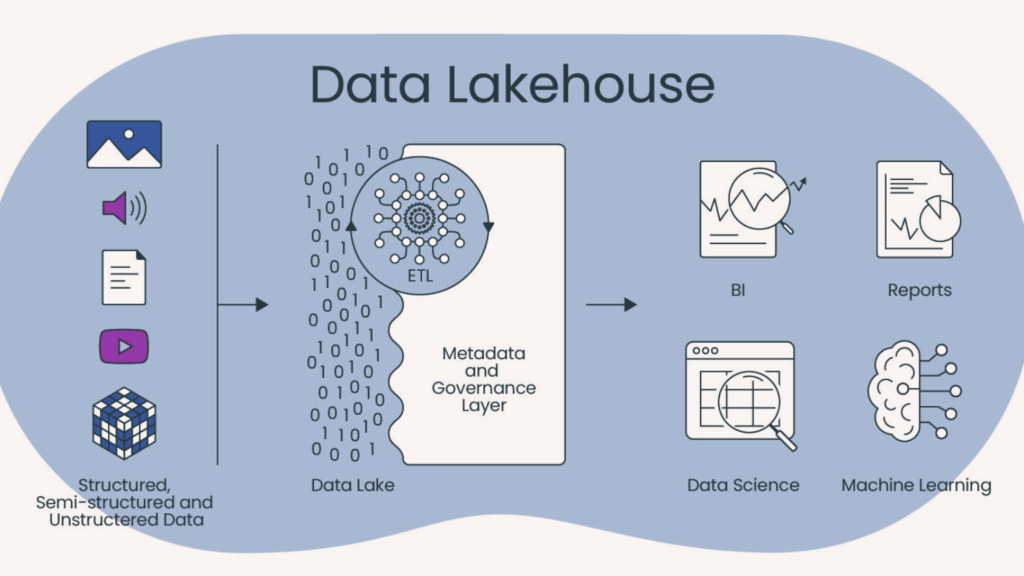
Data Lake vs Lakehouse: What’s the Difference?
The core difference between a Data Lake and a Lakehouse lies in their structure and use cases.
As mentioned earlier, a Data Lake is a vast, unstructured repository. It is most effective when your business requires complex analysis and predictive modeling on raw data. However, its lack of structure can lead to data management challenges. These include data swamps, where data becomes unfindable and unusable.
On the other hand, a Lakehouse, with its structured and unstructured data capabilities, can cater to a broader spectrum of business needs. It integrates the best of Data Lakes and Data Warehouses. Thus, providing a balanced and holistic solution to manage your data. With a Lakehouse, businesses can perform standard reporting, real-time analytics, and advanced data analysis all within a single platform. However, the creation and maintenance of a Lakehouse require more resources and effort.
Lakehouse vs Data Lake: The Right Choice
Choosing between a Data Lake and a Lakehouse largely depends on the specific needs and resources of your organization. If your organization deals with large volumes of unstructured data and requires complex data analysis, a Data Lake would serve you well. Conversely, if your organization seeks a more structured environment for diverse analytics and reporting needs, a Lakehouse would be the more apt choice.
Conclusion
The choice between a Data Lake and a Lakehouse is not a matter of which one is superior. Rather, it’s about identifying the specific needs of your organization and choosing the tool that can best meet those needs. Both offer distinct advantages and understanding these can enable businesses to harness their data effectively. and drive informed decision-making.