Data management is a critical aspect of modern business operations. With the increasing volume and complexity of data, organizations are looking for ways to improve their data management processes. One of the most promising solutions is the use of artificial intelligence (AI) methodologies. In this article, we will explore how AI is improving data management and the benefits it offers.
Data Management
Data management is the process of collecting, storing, organizing, and maintaining data. It involves a range of activities, including data entry, data processing, data analysis, and data visualization. Effective data management is essential for organizations to make informed decisions, improve operational efficiency, and gain a competitive advantage.
However, traditional data management approaches are often time-consuming, error-prone, and limited in their ability to handle large volumes of data. This is where AI comes in. AI methodologies can help organizations automate data management processes, reduce errors, and gain insights from large datasets.
Accelerate Your Career in AI with Best Machine Learning Courses
Challenges in Data Management
Before we dive into how AI is improving data management, let’s first look at some of the challenges organizations face in managing their data.
- One of the biggest challenges is the sheer volume of data. With the rise of big data, organizations are dealing with massive amounts of data that are difficult to manage using traditional methods.
- Another challenge is the complexity of data. Data comes in different formats, from structured data in databases to unstructured data in emails and social media. This makes it difficult to integrate and analyze data from different sources.
Finally, data management is often a manual process that is prone to errors. Data entry errors, for example, can lead to inaccurate data that can have serious consequences for decision-making.
How AI is Improving Data Management ?
AI methodologies are transforming data management by automating processes, reducing errors, and providing insights from large datasets. Here are some of the ways AI is improving data management:
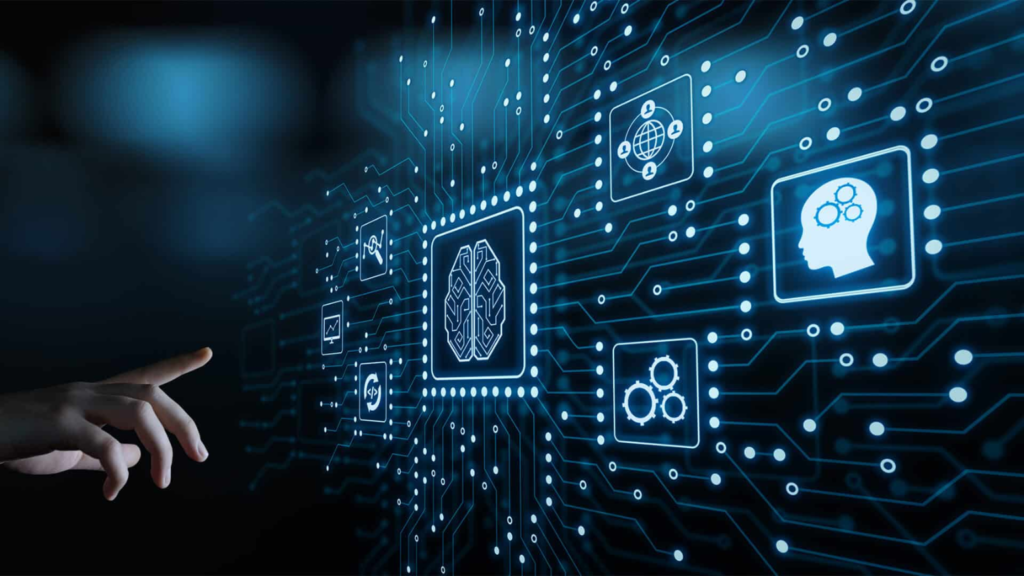
Data Integration
One of the key benefits of AI in data management is its ability to integrate data from different sources. AI algorithms can analyze data from various sources, including structured and unstructured data, and integrate them into a single dataset. This makes it easier for organizations to analyze data and gain insights.
Data Cleansing
Data cleansing is the process of identifying and correcting errors in data. AI algorithms can automate this process by identifying errors and inconsistencies in data and correcting them automatically. This reduces the risk of errors and ensures that data is accurate and reliable.
Predictive Analytics
AI algorithms can analyze large datasets and identify patterns and trends that are difficult to detect using traditional methods. This is known as predictive analytics. By analyzing historical data, AI algorithms can predict future trends and outcomes, enabling organizations to make informed decisions.
Natural Language Processing
Natural language processing (NLP) is a branch of AI that deals with the interaction between computers and human language. NLP algorithms can analyze unstructured data, such as emails and social media posts, and extract valuable insights. This can help organizations understand customer sentiment, identify emerging trends, and improve customer service.
Machine Learning
Machine learning is a subset of AI that involves training algorithms to learn from data. Machine learning algorithms can analyze large datasets and identify patterns and trends that are not immediately apparent. This can help organizations make more accurate predictions and improve decision-making.
Power Your Analytics with the Best Business Intelligence Dataset
Real-World Examples
AI is already being used in a range of industries to improve data management. Here are some real-world examples:
Healthcare
AI is being used in healthcare to improve patient outcomes and reduce costs. For example, AI algorithms can analyze patient data to identify patterns and predict which patients are at risk of developing certain conditions. This can help healthcare providers intervene early and prevent costly hospitalizations.
Finance
AI is being used in finance to improve fraud detection and risk management. For example, AI algorithms can analyze financial transactions and identify patterns that indicate fraudulent activity. This can help financial institutions prevent fraud and reduce losses.
Retail
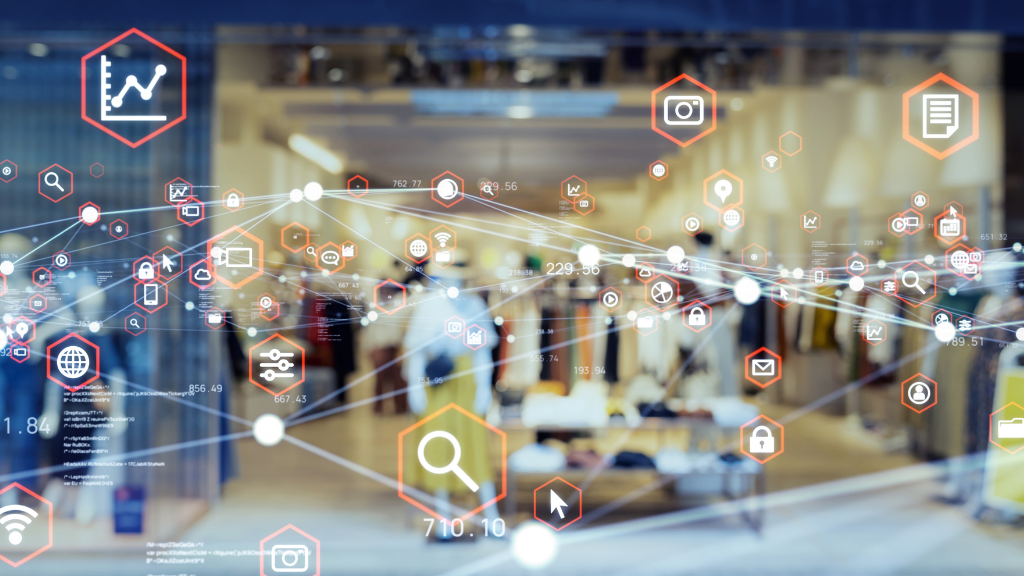
AI is being used in retail to improve customer experience and increase sales. For example, AI algorithms can analyze customer data to identify buying patterns and make personalized product recommendations. This can help retailers improve customer satisfaction and increase sales.
Conclusion
AI is transforming data management by automating processes, reducing errors, and providing insights from large datasets. By integrating AI methodologies into their data management processes, organizations can improve decision-making, reduce costs, and gain a competitive advantage. As AI continues to evolve, we can expect to see even more innovative solutions for data management in the future.