Robotic systems for object handling in warehouses can expedite fulfillment of customer orders by automating tasks such as object picking, sorting, and packing. However, building reliable and scalable robotic systems for object manipulation in warehouses is not trivial. Modern warehouses process millions of unique objects with diverse shapes, materials, and other physical properties. These objects are often stored in unstructured configurations within containers which pose challenges for robotic perception and planning.
From 2015 to 2017, the Amazon Robotics Challenge (ARC) helped push the state-of-the-art for robotic systems in a pick-and-place task representative of a warehouse. In this article, we will explore Amazon’s ARMBench dataset, a large-scale, object-centric benchmark dataset for robotic manipulation in the context of a warehouse.
The Emergence of Get Paid to Do Tasks Copy
ARMBench Dataset Overview
ARMBench contains images, videos, and metadata that corresponds to 235K+ pick-and-place activities on 190K+ unique objects. The data is captured at different stages of manipulation, i.e., pre-pick, during transfer, and after placement. Benchmark tasks are proposed by virtue of high-quality annotations and baseline performance evaluation are presented on three visual perception challenges, namely 1) object segmentation in clutter, 2) object identification, and 3) defect detection. ARMBench can be accessed at http://armbench.com.
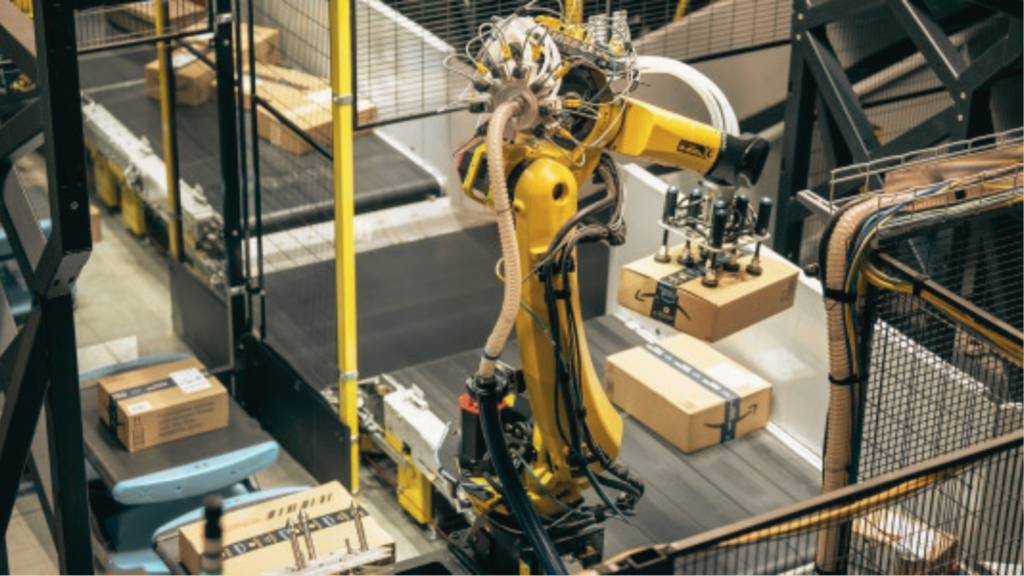
Data Collection
The ARMBench dataset was collected in an Amazon warehouse using a robotic manipulator performing object singulation from containers with heterogeneous contents. The dataset captures diversity in objects with respect to Amazon product categories as well as physical characteristics such as size, shape, material, deformability, appearance, fragility, etc.
The dataset presents a collection of sensor data acquired by a robotic manipulation workcell performing pick-and-place operation, metadata and reference images for objects in containers, a set of annotations acquired either automatically, by virtue of the system design, or via manual labeling, and tasks and metrics to benchmark perception algorithms for robotic manipulation.
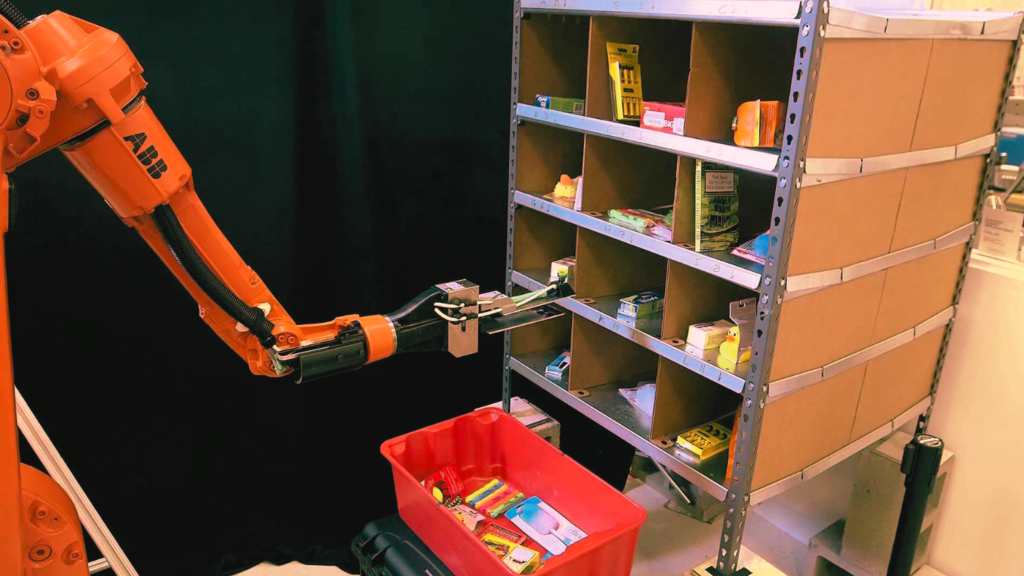
Benchmark Tasks and Annotation Statistics
The ARMBench dataset presents a variety of benchmark tasks and annotation statistics. The left side of Fig. 2 illustrates the benchmark tasks and annotation statistics on the ARMBench dataset. The right side of Fig. 2 shows the distribution of product-groups and object dimensions for 190,00+ unique objects in the dataset. The dataset captures diversity in objects with respect to Amazon product categories as well as physical characteristics such as size, shape, material, deformability, appearance, fragility, etc.
The benchmark tasks proposed in ARMBench are object segmentation, object identification, and defect detection. Object segmentation is the task of identifying the pixels that belong to an object in an image. Object identification is the task of recognizing the object category and instance from an image. Defect detection is the task of identifying defects in objects such as cracks, dents, or deformations. The annotations for these tasks are provided in the form of pixel-level segmentation masks, object category labels, and defect labels, respectively. The dataset also provides metrics to evaluate the performance of algorithms on these tasks, such as mean intersection over union (mIoU) for segmentation, top-1 accuracy for identification, and precision-recall curves for defect detection.
Baseline Performance Evaluation
ARMBench provides baseline performance evaluation for the benchmark tasks on a set of state-of-the-art algorithms. The evaluation is performed on a held-out test set of 10,000 images. The results show that the proposed benchmark tasks are challenging and require further research to achieve high performance. For example, the best-performing algorithm achieves an mIoU of 0.44 on the object segmentation task, which is significantly lower than the state-of-the-art performance on other datasets such as COCO and PASCAL VOC. Similarly, the top-1 accuracy for object identification is 0.54, which is lower than the performance on other datasets such as ImageNet. These results highlight the need for developing algorithms that can generalize to a wide variety of objects and configurations in warehouse environments.
When AI Attracts Attention: Dall E Mini Too Much Traffic
Conclusion
Amazon’s ARMBench dataset is a comprehensive solution for robotic manipulation in warehouses. The dataset captures the diversity of objects and configurations in warehouse environments and provides benchmark tasks and annotations for evaluating visual perception algorithms. The baseline performance evaluation shows that the proposed benchmark tasks are challenging and require further research to achieve high performance. ARMBench is a valuable resource for researchers and practitioners working on robotic systems for object manipulation in warehouses.