A Bayesian Network is also known as a belief network or directed acyclic graphical model. It is a probabilistic graphical model that represents a set of variables and their conditional dependencies via a directed acyclic graph (DAG). In simpler terms, Bayesian networks are mathematical models that represent the relationships among variables. In doing so, it helps in predicting outcomes based on input data.
On the other hand, a Neural Network, inspired by biological neurons, is a computing system. It consists of highly interconnected nodes or “neurons” that process information. They process information by responding to external inputs and relaying information between each unit. Neural Networks are designed to classify and pattern information in a manner similar to the human brain.
So, the key difference lies in their operational mechanisms: Bayesian Networks employ probabilistic relationships. Whereas Neural Networks rely on learning patterns in data through complex, neuron-like structures.
Is a Bayesian Network a Neural Network?
Given their differing natures, it would be incorrect to categorize Bayesian Networks as a type of Neural Network. They are distinct models with unique theoretical foundations. However, in certain situations, the techniques can be merged to create a Bayesian Neural Network, an intriguing fusion that we will discuss later in this article.
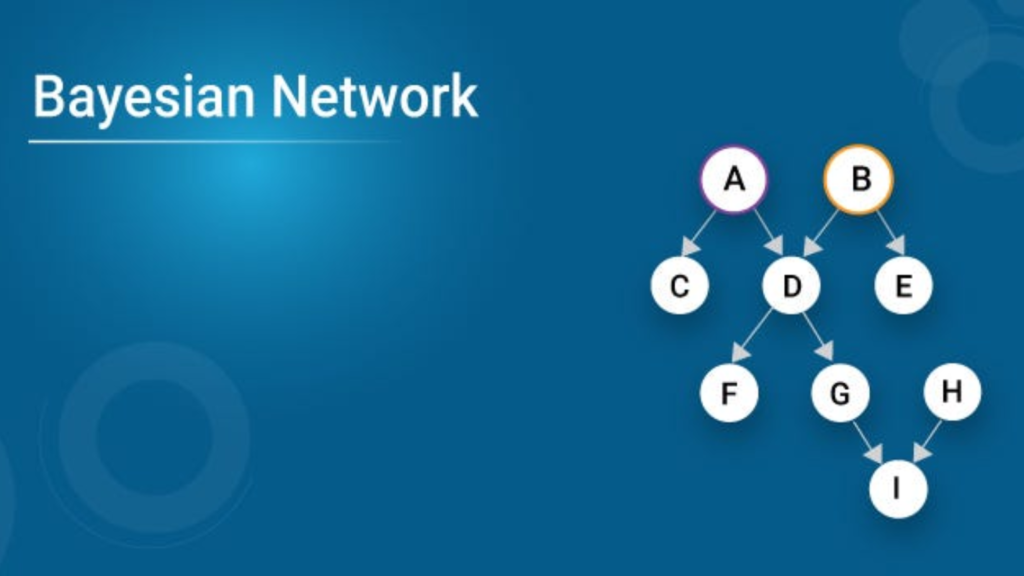
What is the difference between Machine Learning and Bayesian Networks?
Machine Learning is a broad field of study and application that encompasses numerous methods and models, including Bayesian Networks. It aims to build systems that can learn from data, improve over time, and make decisions or predictions. A Bayesian Network, on the other hand, is a specific tool used within the field of machine learning. It is designed to model uncertain knowledge and reasoning.
Electric Car Efficiency vs Gas: A Comprehensive Comparison
What is the difference between a Random Forest and a Bayesian Network?
Random Forests and Bayesian Networks are both powerful machine-learning techniques but have different approaches. A Random Forest is an ensemble learning method that operates by constructing multiple decision trees. It outputs the mode of the classes for classification or mean prediction for regression. It is often used for its simplicity and ability to handle large datasets with high dimensionality.
In contrast, a Bayesian Network is a probabilistic graphical model that uses Bayes’ theorem to infer the probabilities of uncertain events. It shines in applications where relationships among features need to be modeled explicitly or where interpretability is critical.
What is the difference between Naïve Bayes and Bayesian Networks?
Naïve Bayes is a simplified version of a Bayesian Network. In Naïve Bayes, all features are assumed to be independent of each other, an assumption that is “naïve” in many real-world situations. On the contrary, a Bayesian Network represents dependencies among all variables and does not necessarily assume independence, providing a more realistic and complex modeling approach.
Bayesian Neural Network
Now let’s discuss the intriguing hybrid of the two methods we mentioned earlier – the Bayesian Neural Network. This model integrates the complexity and learning capacity of a Neural Network with the probabilistic approach of a Bayesian model.
A Bayesian Neural Network captures the uncertainty in its predictions by providing a distribution of outcomes rather than a single-point estimate. This feature makes Bayesian Neural Networks highly useful in tasks where estimation of uncertainty is crucial. This means you can use it for financial forecasting or medical diagnosis.
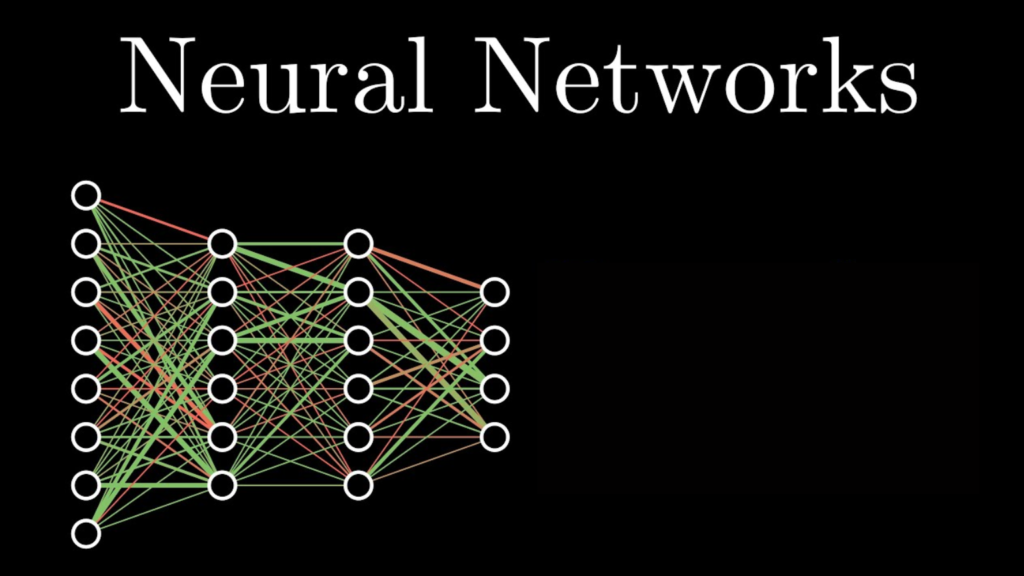
Bayesian Neural Network Example
Consider a Bayesian Neural Network deployed for predicting stock market trends. Unlike a regular Neural Network that might predict a specific value, a Bayesian Neural Network would give a range of potential values with associated probabilities. This feature is particularly useful for traders, who could not only gain insight into expected trends but also understand the inherent uncertainty in these predictions.
Bayesian Neural Network for Classification
In a classification task, a Bayesian Neural Network provides the probabilities for each class, allowing for a more nuanced understanding of the results. This can be particularly useful when working with imbalanced datasets, where misclassification costs may vary among classes.
Bayesian Neural Network Tutorial
Delving into the step-by-step details of building a Bayesian Neural Network is beyond the scope of this article. Resources such as the TensorFlow Probability library provide extensive documentation and tutorials for those interested in implementing this innovative hybrid model.
Conclusion
Bayesian Networks and Neural Networks are distinct models with unique advantages and applications. Their fusion into a Bayesian Neural Network opens up new possibilities for modeling uncertainty in complex predictive tasks. As the field of machine learning continues to evolve, the understanding and application of these models will continue to be an essential aspect of any data scientist’s toolkit.