Image classification is a fundamental task in computer vision that involves assigning a label to an image based on its content. With the increasing availability of digital images, the need for accurate and efficient image classification models has become more important than ever.
Convolutional neural networks (CNNs) have emerged as a powerful tool for image classification, achieving state-of-the-art performance on various datasets. In this article, we will explore the best image classification models based on a survey conducted by Wei Wang, Yujing Yang, Xin Wang, Weizheng Wang, and Ji Li.
We will also compare various image classification methods and present experimental results of different models. Finally, we will highlight the latest innovations in network architecture for CNNs in image classification and discuss future research directions in the field.
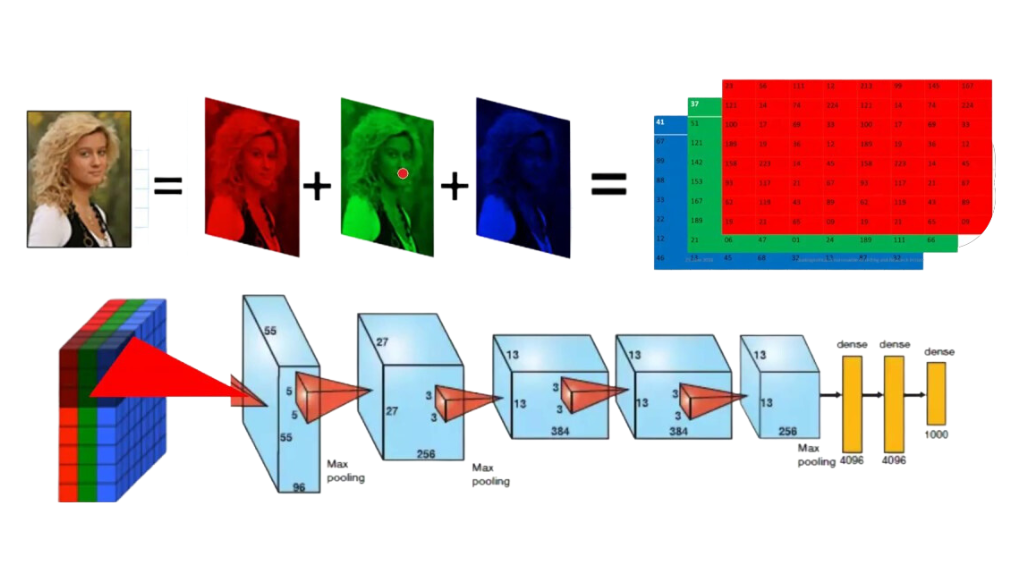
Also Check Will Innovation Save Us From Recession in 2023? | Investing in Generative AI
Best Image Classification Models
1. Sparse coding:
Sparse coding is a method of representing data in a high-dimensional space using a small number of basis functions. In image classification, sparse coding is used to learn a dictionary of basis functions that can be used to represent images. The method involves finding a sparse representation of an image by solving an optimization problem that minimizes the difference between the image and its representation in terms of the learned basis functions. Sparse coding has been used in image classification with some success, but it has been largely superseded by deep learning methods.
2. SIFT + FVs:
Scale-Invariant Feature Transform (SIFT) is a method for detecting and describing local features in images. Fisher Vector (FV) is a method for encoding the distribution of local features in an image. SIFT + FVs is a popular method for image classification that involves extracting SIFT features from an image, encoding them using FVs, and using a classifier to predict the class of the image. SIFT + FVs has been used in several image classification competitions and has achieved state-of-the-art performance on some datasets.
3. AlexNet:
AlexNet is a deep convolutional neural network that was developed by Alex Krizhevsky, Ilya Sutskever, and Geoffrey Hinton. It won the ImageNet Large Scale Visual Recognition Challenge (ILSVRC) in 2012 and was the first deep learning model to achieve state-of-the-art performance on the ImageNet dataset. AlexNet consists of five convolutional layers, followed by three fully connected layers. It uses the ReLU activation function and dropout regularization to prevent overfitting. AlexNet introduced several innovations that are now standard in deep learning, such as the use of GPUs for training and the use of data augmentation to increase the size of the training set.
4. VGGNet:
VGGNet is a deep convolutional neural network that was developed by the Visual Geometry Group at the University of Oxford. It achieved second place in the ILSVRC 2014 competition and has been widely used in image classification tasks. VGGNet consists of 16 or 19 layers of convolutional and fully connected layers. It uses small 3×3 filters in all convolutional layers, which allows it to learn more complex features. VGGNet also introduced the use of batch normalization to improve the training of deep neural networks.
5. GoogLeNet/Inception:
GoogLeNet, also known as Inception v1, is a deep convolutional neural network that was developed by researchers at Google. It won the ILSVRC 2014 competition and introduced the Inception module, which allows the network to learn features at multiple scales. The Inception module consists of parallel convolutional layers with different filter sizes, which are concatenated to form the output of the module. GoogLeNet also introduced the use of global average pooling, which reduces the number of parameters in the network and helps prevent overfitting. The network consists of 22 layers and has a relatively small number of parameters compared to other deep learning models.
6. ResNet:
ResNet, short for Residual Network, is a deep convolutional neural network that was developed by researchers at Microsoft. It won the ILSVRC 2015 competition and introduced the concept of residual connections, which allow the network to learn residual functions instead of directly learning the underlying mapping. Residual connections help prevent the vanishing gradient problem that can occur in very deep neural networks. ResNet consists of many layers, with some versions having over 100 layers. It has been shown to achieve state-of-the-art performance on several image classification tasks.
7. DenseNet:
DenseNet is a deep convolutional neural network that was developed by researchers at Facebook AI Research. It introduces the concept of dense connections, which connect each layer to every other layer in a feed-forward fashion. Dense connections allow the network to reuse features learned in previous layers and can help prevent overfitting. DenseNet consists of several dense blocks, each of which contains several convolutional layers and a transition layer that reduces the spatial dimensions of the output. DenseNet has achieved state-of-the-art performance on several image classification tasks and has been shown to be more parameter-efficient than other deep learning models.
How to Survive the End of the World | Peter Zeihan Latest is Wrong
What are some of the benefits of using CNNs in image classification compared to traditional methods?
CNNs have several benefits over traditional methods for image classification. One of the main benefits is that CNNs can automatically learn features from raw data, whereas traditional methods require hand-crafted features. This means that CNNs can learn more complex and abstract features that are better suited for image classification tasks. Additionally, CNNs can handle images of different sizes and orientations, whereas traditional methods require images to be pre-processed to a fixed size and orientation. CNNs are also more robust to noise and variations in lighting conditions. Finally, CNNs can be trained end-to-end, which means that the entire network can be optimized for the task of image classification, whereas traditional methods require separate optimization of feature extraction and classification.
What is the best Convolutional Neural Network (CNN) for image classification?
There is no single “best” convolutional neural network (CNN) for image classification, as the performance of a CNN depends on several factors, such as the size and complexity of the dataset, the amount of training data, and the computational resources available. However, several CNN architectures have achieved state-of-the-art performance on various image classification tasks. Some of the most popular CNN architectures for image classification include AlexNet, VGGNet, GoogLeNet/Inception, ResNet, and DenseNet. The choice of CNN architecture depends on the specific requirements of the image classification task and the available resources for training and inference.
Can CNNs be applied to other visual recognition tasks beyond image classification?
Yes, CNNs can be applied to other visual recognition tasks beyond image classification, such as object detection, object localization, and semantic segmentation. In fact, the CNN architecture applied to these tasks is generally derived from the network architecture in image classification. For example, in object detection, a CNN can be used to classify objects in an image and localize their positions. In semantic segmentation, a CNN can be used to classify each pixel in an image into different categories. The ability of CNNs to automatically learn features from raw data and handle the spatial structure of images makes them well-suited for a wide range of visual recognition tasks.