Generative AI has been a rapidly growing field in recent years, with text-to-image synthesis being one of the most exciting and promising areas of research. In this article, we review the latest developments in diffusion model image generation, which have emerged as a popular approach for a wide range of generative tasks.
What Is a Diffusion Model? How Does It Work for Image Synthesis
Diffusion models (DMs) are a family of generated models that are Markov chains trained with variational inference. The learning goal of DM is to reserve a process of perturbing the data with noise, i.e. diffusion, for sample generation. As a milestone work, the denoising diffusion probabilistic model (DDPM) was published in 2020 and sparked an exponentially increasing interest in the community of generative models afterward.
The PDF provides a self-contained introduction to DDPM by covering the most related progress before DDPM and how unconditional DDPM works with image synthesis as a concrete example. Moreover, the PDF summarizes how guidance helps in conditional DM, which is an important foundation for understanding text-conditional DM for text-to-image.
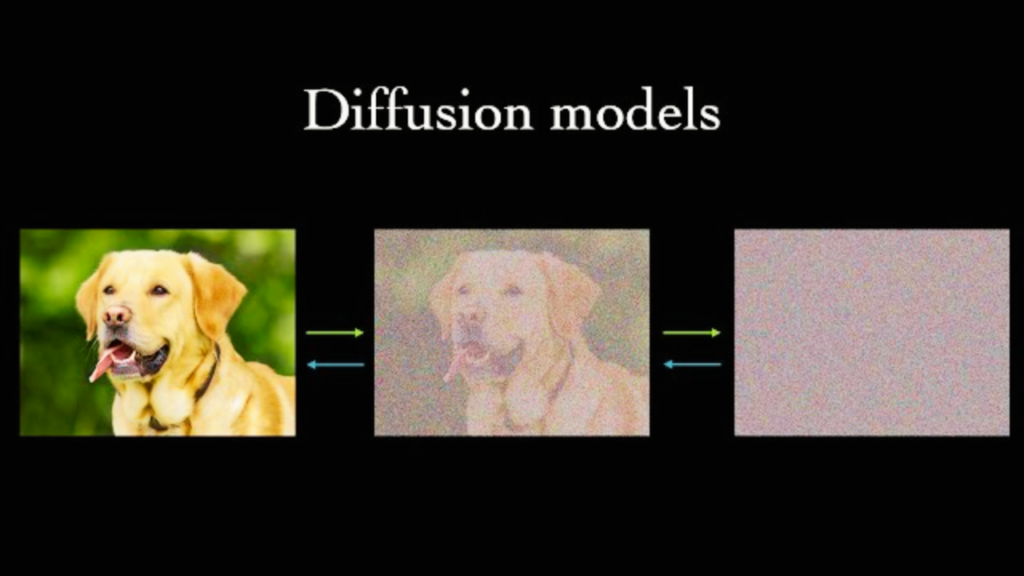
What Diffusion Model Image Generation Is All About?
Diffusion model image generation is a process of perturbing the data with noise, i.e. diffusion, for sample generation. According to 2, diffusion models (DMs) are a family of generated models that are Markov chains trained with variational inference. The learning goal of DM is to reserve a process of perturbing the data with noise, i.e. diffusion, for sample generation. The denoising diffusion probabilistic model (DDPM) is a type of diffusion model that generates images from noise within finite transitions during inference. DDPMs are defined as a parameterized Markov chain. The PDF provides a self-contained introduction to DDPM by covering the most related progress before DDPM and how unconditional DDPM works with image synthesis as a concrete example.
Text-Conditioned Models Improve Learning in Image Generation
Text-conditioned models improve learning in image generation by providing guidance to the generative model. Specifically, text-to-image generation is a task where a generative model is conditioned on a text description to generate a corresponding image.
The text description provides guidance to the generative model, which helps it to generate more realistic and accurate images. Text-conditioned models use diffusion models (DMs), which are a family of generated models that are Markov chains trained with variational inference. The learning goal of DM is to reserve a process of perturbing the data with noise, i.e. diffusion, for sample generation. Text-conditioned DMs use the text description as a condition to guide the diffusion process, which improves the quality of the generated images.
Existing Challenges and Promising Future Directions in Text-To-Image Generation
Certainly! Here are the subheadings for the existing challenges and promising future directions in text-to-image generation:
Existing Challenges
1: Limited Dataset Availability:
One of the main challenges in text-to-image generation is the limited availability of large-scale datasets that contain both text descriptions and corresponding images.
Datacamp vs Codecademy: Which Platform is Right for You?
2: Ambiguity in Textual Descriptions:
Textual descriptions can be ambiguous and subjective, which makes it difficult for generative models to accurately interpret and generate corresponding images.
3: Complex Scene Understanding:
Generating realistic images from textual descriptions requires a deep understanding of complex scenes, including object relationships, spatial layouts, and lighting conditions.
Promising Future Directions
1: Multi-Modal Learning:
Multi-modal learning is a promising direction for improving text-to-image generation. This approach involves training generative models on multiple modalities, such as text, images, and audio, to improve their ability to generate realistic images from textual descriptions.
2: Attention Mechanisms:
Attention mechanisms can help generative models focus on specific parts of the text description that are most relevant to generating a corresponding image. This approach can improve the accuracy and realism of the generated images.
Kaggle vs Jupyter: Which One Suits Your Data Science Needs
3: Incorporating External Knowledge:
Incorporating external knowledge, such as object recognition and scene understanding, can help generative models better understand and interpret textual descriptions, leading to more accurate and realistic image generation.
Overall, while there are still challenges to overcome in text-to-image generation, promising future directions such as multi-modal learning, attention mechanisms, and incorporating external knowledge offer exciting opportunities for improving the accuracy and realism of generated images.
Final Thoughts!
In conclusion, text-to-image generation is a fascinating and challenging task in the field of generative AI. While there are still challenges to overcome, such as limited dataset availability and ambiguity in textual descriptions, promising future directions such as multi-modal learning, attention mechanisms, and incorporating external knowledge offer exciting opportunities for improving the accuracy and realism of generated images. As we continue to make progress in this field, we may one day be able to create generative models that can generate visually realistic images from textual descriptions with human-like accuracy and creativity. The possibilities are endless, and I am excited to see what the future holds for text-to-image generation and generative AI as a whole.