In the realms of technology and innovation, we often find ourselves marvelling at the strides made by Artificial Intelligence (AI). But what if we told you that AI is not only revolutionizing industries but is also capable of creating its own kind.How AI Can Create AI.
Yes, you read that right! The concept of AI creating AI might seem like a scene out of a science fiction movie, but it’s a fascinating reality that’s shaping the future of technology.
Overfitting vs Underfitting: Difference Explained
How AI Can Create AI
At its core, AI creating AI involves leveraging machine learning techniques to automate and enhance the process of AI development. This iterative and self-improving mechanism is a testament to the remarkable capabilities of modern AI systems. But how exactly does this intriguing phenomenon work?
Imagine a scenario where a machine learning model, often referred to as a “parent” model, is trained on vast amounts of data, including programming languages, algorithms, and patterns. This parent model becomes proficient in understanding the intricacies of code and problem-solving methodologies. With this knowledge, it can then generate a new model, known as the “child” model, which is designed to perform specific tasks or solve certain problems.
Google’s artificial intelligence has accomplished a remarkable feat – crafting an AI that surpasses the capabilities of any human-made counterpart.
In a groundbreaking revelation, Google Brain’s researchers have unveiled the dawn of a new era in artificial intelligence (AI). Enter AutoML, an ingenious creation capable of generating its very own AI offspring. But that’s not all – in a recent demonstration of its prowess, AutoML’s prodigy managed to outshine its human-crafted counterparts in a remarkable display of technological advancement.
The innovation at Google took shape through the orchestration of machine learning models, choreographed by the principles of reinforcement learning. Acting as a conductor in this intricate symphony, AutoML emerged as a controller neural network with the innate ability to give birth to child AI networks, each designed for specific tasks.
One of these AI children, christened NASNet, embarked on the challenge of real-time object recognition in videos, deciphering the presence of objects ranging from people and cars to traffic lights, handbags, and backpacks.
The process that unfolded was a cascade of learning, where AutoML meticulously evaluated NASNet’s performance and employed this information to refine and enhance its offspring AI. This cyclic refinement was repeated thousands of times, each iteration honing the child AI’s abilities with precision.
When put to the test against the formidable ImageNet image classification and COCO object detection datasets – lauded as “two of the most respected large-scale academic datasets in computer vision” by the Google researchers – NASNet transcended expectations. Its predictive accuracy soared to an impressive 82.7 percent on ImageNet’s validation set, outperforming previously published results by a staggering 1.2 percent. The brilliance of the system extended further as it showcased a 43.1 percent mean Average Precision (mAP), boasting an efficiency boost of 4 percent.
Remarkably, the reign of NASNet wasn’t confined to power-intensive setups alone. Even a lighter, less computationally demanding version of NASNet showcased its might by surging ahead of comparably sized models for mobile platforms by an impressive 3.1 percent.
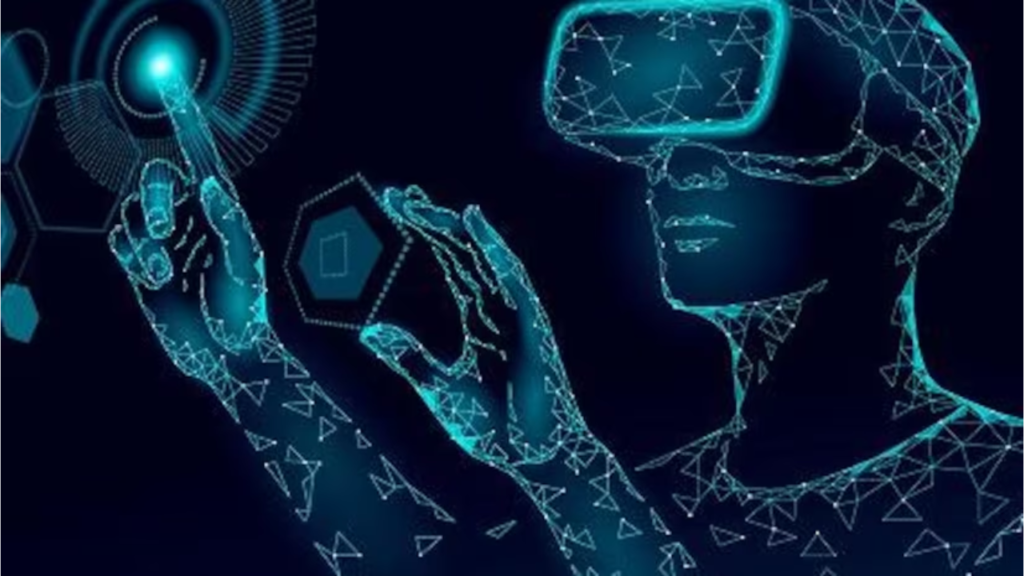
The Mechanics in Action
The process of AI creating AI involves several fascinating components that work in harmony:
1: Data Ingestion
The parent AI model consumes large datasets, including source code repositories, programming manuals, and problem-solving scenarios. This data provides the foundation for understanding programming syntax, logic, and common challenges.
2: Learning and Iteration:
Through a combination of neural networks and deep learning algorithms, the parent AI model learns the underlying patterns and structures of programming. It’s able to generate code, suggest optimizations, and even identify potential bugs.
3: Generation of Child Models:
With its newfound proficiency, the parent AI model generates child AI models that are tailored to specific tasks or domains. For instance, it can develop models focused on image recognition, language translation, or even code generation.
4: Fine-Tuning and Validation:
The child AI models are fine-tuned and validated against benchmark datasets to ensure their accuracy and reliability. This phase ensures that the generated AI is effective and capable of delivering the desired outcomes.
Real-World Examples
The concept of AI creating AI has already manifested in various projects with promising results:
Google AutoML: Google’s AutoML is a prime example of AI-generated AI. It allows users with limited machine learning expertise to create custom machine learning models. AutoML essentially automates the process of designing and training AI models, making it accessible to a broader audience.
OpenAI’s GPT-3: OpenAI’s GPT-3, a groundbreaking language model, can generate code snippets based on natural language prompts. While not exactly AI creating AI, it showcases AI’s ability to assist in the coding process, blurring the lines between human and AI-driven creativity.
The Road Ahead
As AI continues to evolve, the concept of AI creating AI holds immense promise. It has the potential to accelerate innovation, reduce the barrier to entry for AI development, and pave the way for new breakthroughs across industries. However, this phenomenon also raises important questions about ethical considerations, human oversight, and the future role of developers in an AI-dominated landscape.
Wrap Up:
In conclusion, the concept of AI creating AI is a testament to the incredible advancements we’ve made in the world of technology. It’s a reminder that innovation knows no bounds, and the lines between creator and creation are becoming increasingly blurred. As we journey into a future where machines play an active role in shaping their own kind, we must navigate the ethical complexities and opportunities that this brave new world presents.