Weights & Biases (W&B) is a cutting-edge platform designed for machine learning practitioners, streamlining the process of tracking and optimizing experiments. This post explores how W&B is revolutionizing the ML workflow.
Background Story
Weights & Biases is a machine learning development platform that provides enterprise-grade, end-to-end MLOps (Machine Learning Operations) workflow to accelerate ML activities. The company was founded in 2017 by Lukas Biewald, Chris Van Pelt, and Shawn Lewis. Lukas Biewald, the CEO of Weights & Biases, is a well-known figure in the machine learning community.
He previously co-founded CrowdFlower, a data enrichment platform, which was later acquired by Figure Eight. Chris Van Pelt, the CTO of Weights & Biases, has a Ph.D. in computer science from UC Berkeley and has worked on several machine learning projects. Shawn Lewis, the COO of Weights & Biases, has a background in finance and has worked in various startups.
Target Customers
Weights & Biases targets companies and individuals who work with machine learning models. The platform is designed to help data scientists, machine learning engineers, and researchers build better models faster. Weights & Biases is used by companies in various industries, including:
- Autonomous vehicles
- Financial services
- Scientific research
- Communications
- Media & entertainment
- Public sector
Featured Customers
Weights & Biases has several high-profile customers, including OpenAI, Toyota Research Institute, NVIDIA, and Google.
- OpenAI, a leading AI research organization, uses Weights & Biases to track and visualize their machine learning experiments.
- Toyota Research Institute uses Weights & Biases to accelerate their autonomous vehicle research.
- NVIDIA, a leading graphics processing unit (GPU) manufacturer, uses Weights & Biases to optimize their deep learning models.
- Google uses Weights & Biases to track and analyze their machine learning experiments.
Funding, Capital Raised, Estimated Revenue
Weights & Biases has raised $250M over 7 rounds of funding. The latest funding round was a Series C – II for $50M on August 9, 2023. Weights & Biases’ valuation in October 2021 was $1,000M. The company has not disclosed its estimated revenue.
What is App Academy? The Courses they offer, Competitors and More
Products and Services
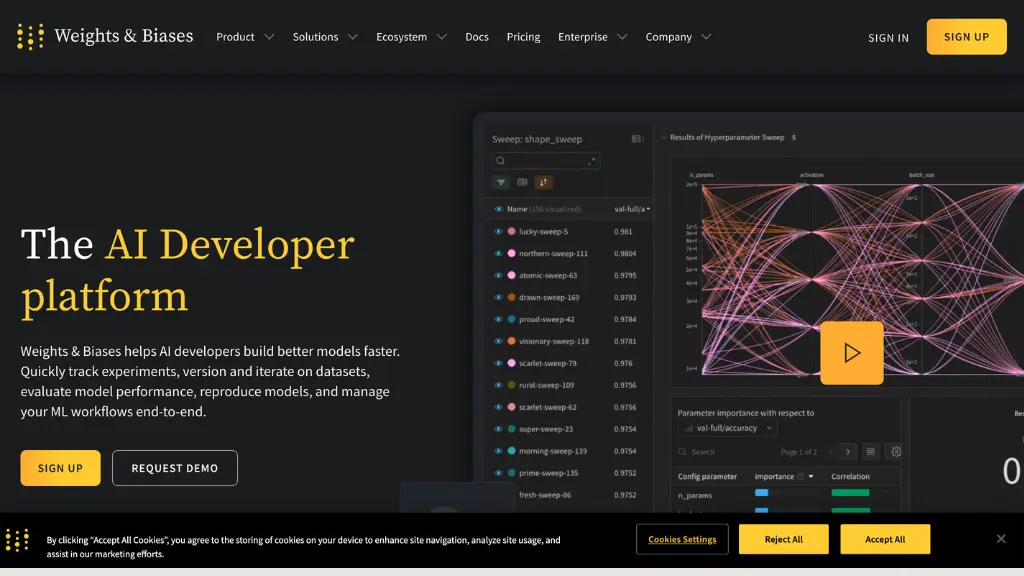
Weights & Biases offers several products and services to help data scientists and machine learning engineers build better models faster. The main products and services are:
W&B MODELS
- Experiments
- Lightweight experiment tracking
- Models Registry
- Centralized model registry
- Launch
- Automated ML workflows
- Sweeps
- Hyperparameter optimization
W&B PROMPTS
- Prompts
- LLMOps and prompt engineering
W&B PLATFORM
- Artifacts
- Dataset and model versioning
- Tables
- Interactive data visualization
- Reports
- Collaborative dashboards
- Weave
- Interactive ML app builder
W&B MONITORING
- Monitoring
- Observability for production ML
Competitors
Weights & Biases faces competition from several other machine learning development platforms, including:
- Comet.ml
- neptune.ai
- Databricks Lakehouse Platform
- SAP HANA Cloud
- ClearML
- Vertex AI
- Determined AI
- IBM Watson Studio
- Saturn Cloud
- SuperAnnotate
Pros and Cons of W & B
Pros
- User-Friendly Interface: W&B is intuitive and easy to use, making it suitable for both beginners and experienced ML practitioners.
- Performance Monitoring: Enables monitoring the effects of architecture changes and hyper-parameter tuning.
- Training Debugging: Helps in identifying and resolving training problems, such as hardware issues related to CUDA and CPU.
- System Dashboard: Assists in optimizing hardware resources, like VRAM usage, to determine the right batch size.
- Variety of Plotting Options: Provides numerous plotting options for better visualization and comparison of models and hyper-parameters.
- Constant Evolution: W&B is continuously updated to stay aligned with the advancements in AI.
- Educative Blog Posts: Their blogs are rich in content and beneficial for gaining ML knowledge.
- Easy Integration: W&B seamlessly integrates with popular frameworks like TensorFlow and allows easy metric tracking per epoch.
- Automatic Metrics Collection: Reduces the manual effort of managing weights, loss, and accuracy charts.
- Collaboration: Facilitates team collaboration, sharing of ML experiments, and maintaining records, promoting best practices.
- Customizable Visualizations: Offers the ability to create custom visualizations using Vega for more nuanced analyses.
- On-Premise Solution: Allows for internal data management by providing an on-premise solution.
- Extensive Features: Offers features like artifact management, hyperparameter sweep, and more for comprehensive ML Ops.
- Accelerates Workflow: Biases engineers towards better practices and simplifies experimental management.
- Real-Time Collaboration: Allows for synchronous collaboration on experiments.
Cons
- UI Delays: The user interface has a slight delay in updating the progress of training, which can be bothersome for some users.
- Limited Features: Users desire additional features like estimated training completion time and better time scales on plots.
- Confusing Analytics Charts: Some charts and default names can be confusing and not immediately intuitive.
- No Model Deployment Functionality: Users would prefer if W&B had a feature to deploy ML models directly.
- Team API Key Limitations: A more flexible system for team API keys is desired for better access control and management.
- Maintenance in Cloud: On-premise solution requires maintenance when deployed in the cloud environment.